
Machine learning in intercoms dramatically improves communication systems by enhancing real-time interactions and personalization. It employs natural language processing (NLP) to understand and respond to user queries, streamlining operations and boosting satisfaction.
Smart chatbots provide instant responses, addressing routine inquiries and freeing up human agents for complex tasks. Additionally, predictive analytics anticipate user needs, offering insights into user behavior and trends. This leads to more informed development and personalized user experiences. Machine learning also supports scalability, maintaining efficiency during peak times.
Understanding these features can reveal the transformative impact machine learning has on traditional communication systems. For instance, our company's Netcam Studio project demonstrates how technological advancements can revolutionize existing systems. By replacing WebcamXP, one of the first video surveillance applications established in 2003, Netcam Studio showcased significant improvements through its reworked web interface in 2013. This project, which involved careful analysis, planning, and wireframing, exemplifies how updating and enhancing user interfaces can greatly improve the functionality and user experience of communication systems.
Key Takeaways
- Machine learning enables smart chatbots, providing instant responses and reducing wait times in intercom systems.
- Predictive analytics in machine learning anticipates user needs, enhancing personalized interactions in intercom systems.
- AI-driven communication powered by machine learning improves the accuracy of real-time user inquiries in intercom systems.
- Machine learning facilitates resolution automation, efficiently handling repetitive issues in intercom communication.
- Voice and emotion recognition, enhanced by machine learning, improves user interaction quality in intercom systems.
Understanding Machine Learning in Intercom
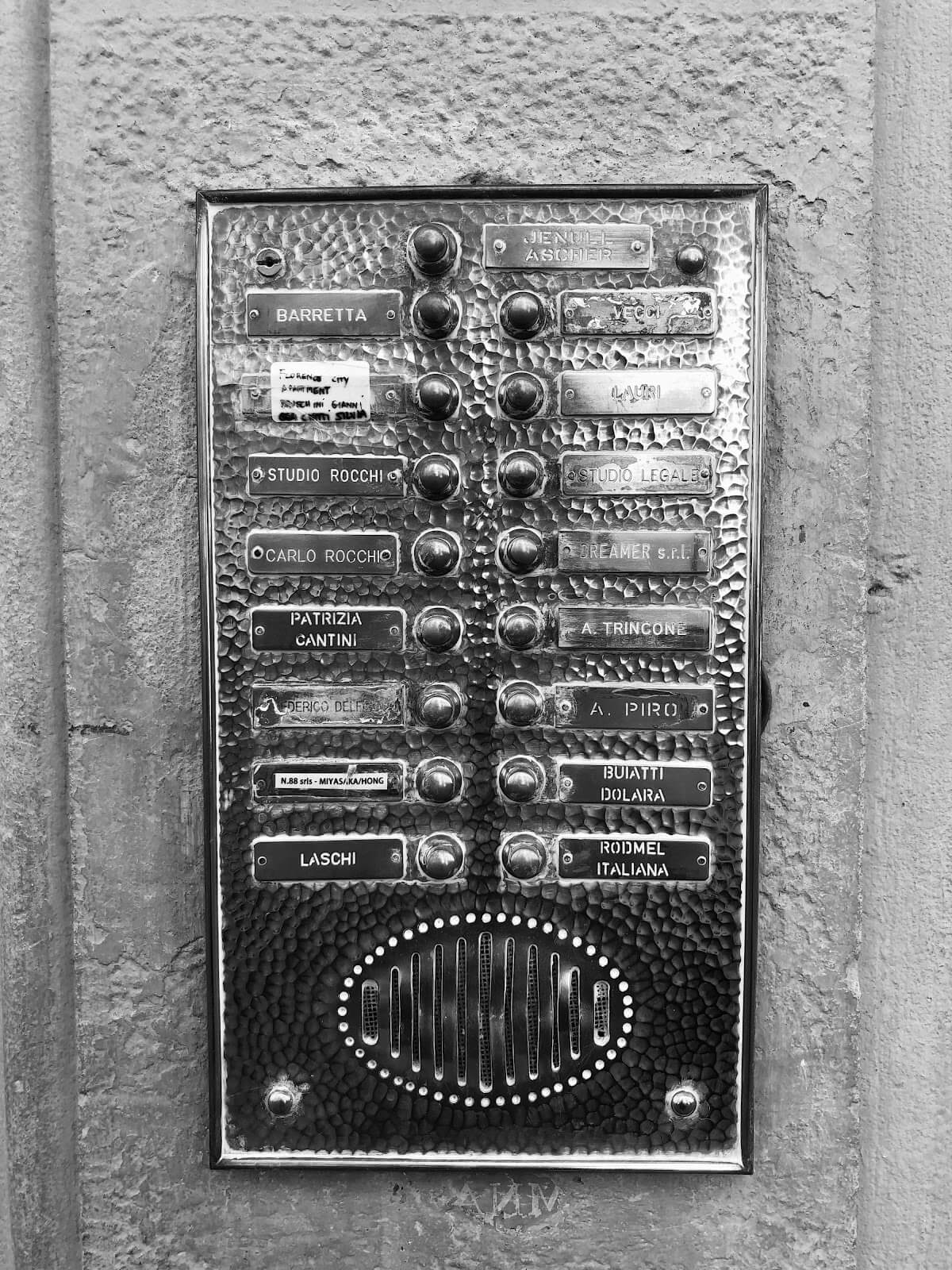
When exploring machine learning in intercom systems, you'll encounter core concepts like supervised, unsupervised, and reinforcement learning, each offering unique ways to enhance communication.
Machine learning plays an essential role in modern communication platforms by enabling features like voice recognition, noise reduction, and predictive maintenance.
By understanding these concepts, you can better integrate machine learning to improve the user experience and operational efficiency of your intercom products.
Core Concepts
Machine learning has become an essential part of modern intercom communication systems, offering a range of capabilities that enhance user interaction and system efficiency. By integrating machine learning in intercoms, you can utilize natural language processing to understand and respond to user queries, improving communication quality.
Reinforcement learning enables these systems to evolve based on user interactions, enhancing algorithm performance over time. This flexibility supports the development of ML-powered features that can predict and address user needs, leading to a more intuitive experience.
By focusing on these core concepts, you guarantee that your intercom system remains competitive, providing users with a seamless, responsive platform. Understanding these machine learning types helps you make informed decisions about integrating them into your product development strategy.
Role of ML in Modern Communication Platforms
Open new dimensions of user engagement by utilizing the strength of machine learning in modern communication platforms. You can utilize AI-driven communication software to improve real-time communication AI, helping customer support teams respond more effectively.
By incorporating advanced language models, your system can understand and process user inquiries with higher accuracy, providing quicker solutions. The integration of production ML infrastructure guarantees the system's scalability and strength, allowing seamless communication flow even during peak times.
As a product owner, employing these technologies enables you to enhance user satisfaction and streamline operations. By adopting machine learning tools, your platform stays competitive, delivering a superior communication experience. This approach not only boosts efficiency but also creates a more responsive and intuitive user interface.
Key ML Features in Intercom Systems
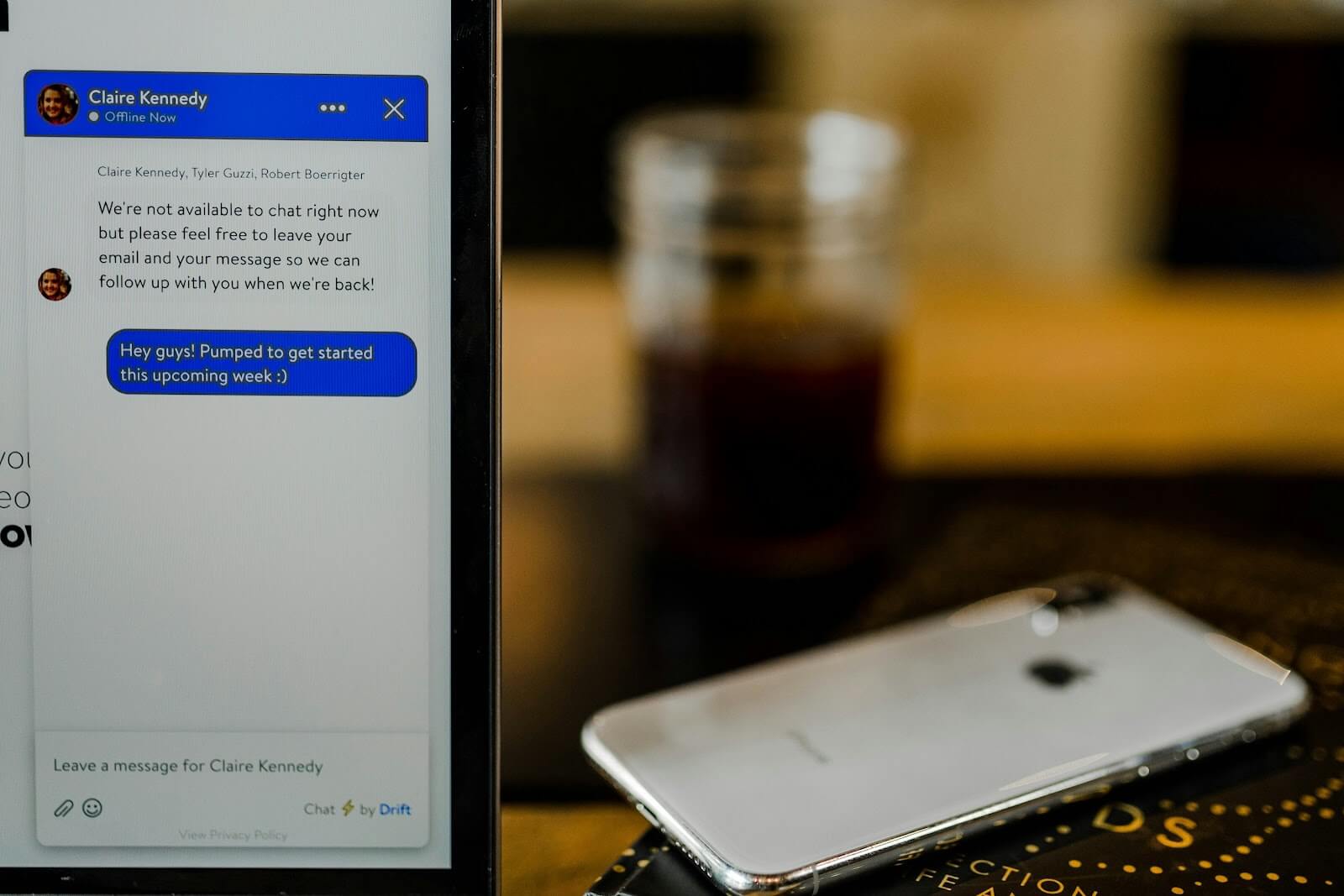
Incorporating machine learning into intercom systems offers product owners a suite of features that can greatly enhance user experience. Smart chatbots and resolution automation streamline support interactions, while predictive analytics provide useful customer understandings that can inform future product development.
Additionally, natural language processing and voice and emotion recognition technologies enable more intuitive and personalized communication, paving the way for more responsive and flexible intercom solutions.
Smart Chatbots and Resolution Automation
Utilizing the strength of smart chatbots and resolution automation can greatly enhance the efficiency of intercom communication systems. By implementing smart chatbots, you can provide instant responses to customer questions, reducing wait times and improving user satisfaction. These chatbots efficiently handle routine inquiries, allowing your engineering teams to focus on more complex issues. The implementation of AI chatbots can lead to a significant increase in customer satisfaction levels, as they provide timely and relevant information to users (Wahab, 2023).
Resolution automation further optimizes the process by automatically addressing repetitive problems, freeing up resources for more critical tasks. This approach doesn't just streamline operations; it allows you to scale data analyses, leading to continuous improvements in service delivery.
By integrating these technologies, you create a more agile and responsive system that adjusts to customer needs, enhancing overall performance and setting the stage for future advancements.
Predictive Analytics and Customer Insights
Predictive analytics offers a potent tool for enhancing intercom communication systems. By utilizing predictive analytics, you can access significant customer knowledge, allowing you to anticipate user needs and improve service delivery. This approach promotes real customer impact by identifying trends and patterns in user behavior, which can guide product development decisions.
Incorporating predictive analytics into your intercom system involves focusing on model development and features in production. This helps guarantee that the system is not only reactive but also proactive in understanding customer requirements. By doing so, you enhance your ability to deliver tailored experiences, ultimately boosting user satisfaction and loyalty. Embrace these technologies to stay ahead in today's competitive market.
Natural Language Processing Capabilities
Natural language processing (NLP) capabilities revolutionize intercom systems by enhancing communication efficiency and user satisfaction. By integrating NLP, you're enabling intercom systems to understand and process human language, which transforms customer interaction.
Machine learning plays a pivotal role, allowing systems to adjust and learn from every conversation, leading to a deep knowledge of user needs and preferences. This results in more personalized and exceptional service, as the intercom can respond more naturally and accurately to inquiries. NLP capabilities also streamline operations by automating responses to common questions, freeing up human agents for more complex tasks.
As a product owner, utilizing these capabilities helps you create a smarter, more responsive intercom system that meets modern user demands effortlessly.
Voice and Emotion Recognition Technology
Voice and emotion detection technology can considerably enhance the functionality of intercom systems, providing a more intuitive and responsive user experience. By integrating these features, you enable millions of users to communicate more effectively, identifying emotional cues and adjusting responses accordingly.
Utilizing advanced unsupervised clustering, these systems can learn from diverse interactions, refining their ability to identify emotions over time. This not only helps in understanding real customer needs but also guarantees that your intercom system responds with strong communication skills.
As a product owner, you'd be offering an advanced solution that modifies to users' emotional states, creating a seamless interaction. This technology can transform how your users engage with the intercom, moving beyond simple voice transmission to a more empathetic communication tool.
Implementation Guide for Product Owners
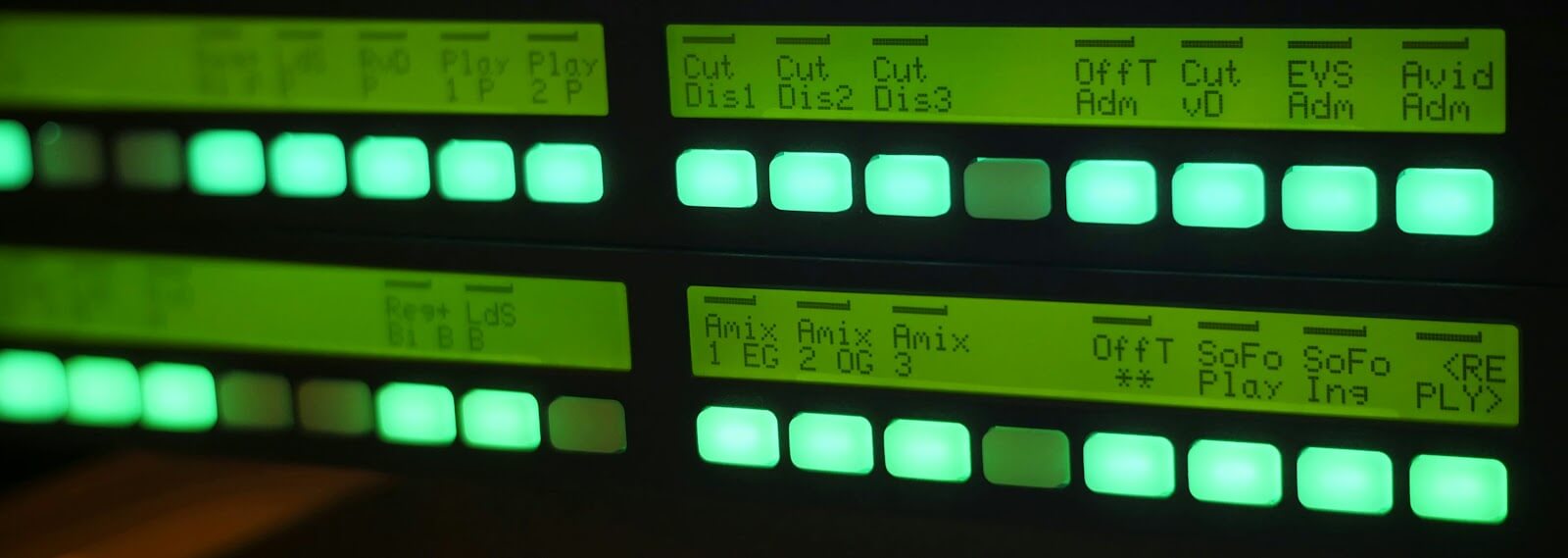
As a product owner, you'll want to focus on effective integration strategies and best practices to seamlessly incorporate machine learning into your intercom systems, ensuring they align with your product goals and user needs.
Measuring success and performance metrics becomes vital, as these will help you assess the impact of these technologies and make data-driven decisions to enhance user experience.
Balancing AI with human support teams is also essential, allowing you to maintain a high standard of customer service while utilizing the efficiency and understanding provided by machine learning.
Integration Strategies and Best Practices
When integrating machine learning into intercom communication systems, it's essential to prioritize strategies that enhance functionality while preserving a seamless user experience. Begin by aligning neural networks with customer expectations to ensure the solution addresses their needs effectively. Implementing machine learning algorithms will help personalize and optimize interactions, such as predicting user intent and providing tailored responses.
Design experiments and A/B testing to allow for continuous improvements, which helps refine the integration strategy over time. This iterative approach ensures that machine learning models evolve to better serve users' needs, optimizing the overall communication experience. According to a study by Akilimalissiga and Sukdeo (2023), implementing A/B testing in machine learning systems can lead to a 20% increase in user satisfaction by tailoring responses based on real-time feedback. This highlights the importance of continuous refinement in enhancing the effectiveness of machine learning integration.
Additionally, explore how machine learning can enhance external communications by automating responses for common queries, improving message clarity, and even detecting sentiment to adjust the tone of interactions. These capabilities not only reduce response times but also create more efficient, intuitive user interactions—ultimately elevating the value of your intercom system.
Here's a short guide to help you:
- Align neural networks with customer expectations to deliver relevant features.
- Design experiments to iteratively test and refine machine learning models.
- Optimize external communications by incorporating automated, intelligent responses.
- Continuously evaluate integration strategies to verify they meet evolving user needs.
Measuring Success and Performance Metrics
To effectively measure success in integrating machine learning (ML) with intercom communication systems, it's essential to establish clear performance metrics that align with your business goals from the outset. Organizations that define clear performance metrics from the outset of integrating ML into intercom systems can achieve improvements in user engagement (Morrison et al., 2021).
Begin by defining metrics that reflect how well your ML solutions are enhancing user interactions. These could include improvements in response times, user engagement, or the system's ability to predict and adapt to user behavior. Incorporating unsupervised clustering techniques can help identify data patterns and optimize system responses, ensuring the intercom system becomes more intuitive over time.
Maintain a balance between innovation and practical functionality by leveraging sound engineering principles. While ML offers the potential for transformative capabilities, it's important to remain adaptable as the outcomes can sometimes be unpredictable. Regularly assess your performance metrics to ensure that your system's integration is meeting expectations and making meaningful contributions to your broader strategic objectives.
By consistently reviewing these metrics and making data-driven adjustments, you can measure the success of ML integration and ensure that it drives improved performance, user satisfaction, and business growth.
Balancing AI and Human Support Teams
While integrating machine learning into intercom communication systems, it's crucial to strike a balance between AI-driven interactions and human support teams to enhance user satisfaction.
By addressing customer issues effectively, you can create a cohesive work environment that supports a protected basis for your team. Implementing a hybrid working policy guarantees your human support team remains flexible and versatile.
To achieve this balance, consider the following:
- Identify Tasks for AI: Use machine learning for repetitive tasks, freeing up your human team for complex issues.
- Seamless Integration: Ascertain AI and human interactions flow smoothly, providing consistent user experiences.
- Continuous Training: Regularly train your team on AI updates to maintain support quality.
- Feedback Loop: Regularly collect feedback from both customers and support teams to fine-tune the balance.
Ethical Considerations and Data Management
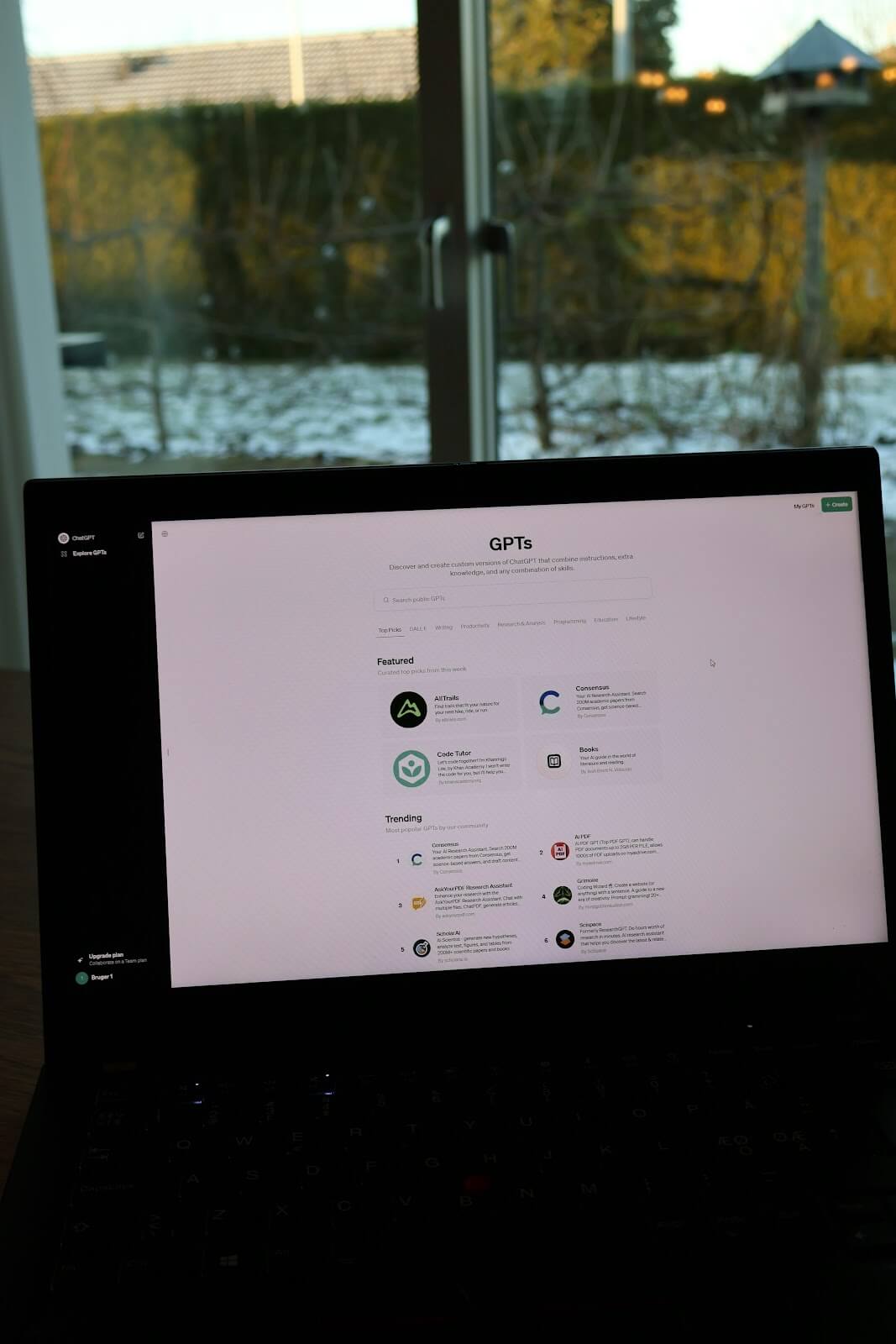
As a product owner, you should focus on developing responsible AI frameworks that prioritize ethical considerations in your intercom communication systems. By training with diverse datasets, you can guarantee your AI models are inclusive and reduce biases, leading to a more equitable user experience.
Additionally, implementing real-time feedback mechanisms allows for continuous improvement and accountability, helping you address any potential ethical issues promptly.
Developing Responsible AI Frameworks
Crafting responsible AI frameworks demands a keen focus on ethical considerations and strong data management practices. As you develop machine learning solutions, it's essential to align them with core values. Product engineers should highlight inclusivity, guaranteeing that systems respect all users, regardless of gender identity.
A sturdy framework not only enhances user trust but also attracts top talent, offering a competitive salary as a reflection of the organization's commitment to ethics.
To guide your efforts, consider the following:
- Establish clear ethical guidelines: Define your core values and make sure they permeate all AI-related activities.
- Prioritize transparent data practices: Be open about data collection, usage, and storage.
- Foster a culture of accountability: Encourage responsibility at every level.
- Regularly review AI outcomes: Continuously assess for biases and inaccuracies.
Training With Diverse Datasets
Utilizing diverse datasets is vital for constructing strong machine learning models that are both effective and fair.
When you train with diverse datasets, you guarantee that your model can handle various scenarios and user demographics, which is essential in an intercom communication system. The functions of teams involved in data management should include gathering data from multiple sources and confirming it's representative of all potential users.
Incorporating diverse datasets improves the reliability of machine learning models in a production environment. Practical stats knowledge is necessary to analyze these datasets effectively, making certain that your models are both unbiased and sturdy. By doing so, you enhance the overall user experience and maintain ethical standards in AI development.
Real-Time Feedback Implementation
Real-time feedback implementation in intercom communication systems can considerably boost user engagement and satisfaction. By integrating this feature, you can enhance product and design decisions, catering to millions of customers effectively.
Consider these key points for successful implementation:
- Identify User Needs: Understand both work and non-work related topics that resonate with your audience.
- Data Privacy: Guarantee data collected from interactions, such as secure bike storage queries, is handled ethically and securely.
- Iterative Design: Use feedback to continually refine your product, addressing user concerns promptly.
- Scalability: Ensure your system can handle a growing number of users without compromising performance.
These strategies can help you maintain a responsive and customer-centric intercom communication system.
Advanced Customer Engagement Strategies
As product owners, you can utilize machine learning to enhance customer engagement by utilizing ML-powered journey mapping, which helps in understanding and optimizing the user path through your product.
By integrating personalization techniques, you guarantee a tailored user experience that resonates with individual preferences, thereby increasing satisfaction and loyalty.
Additionally, cross-platform integration allows you to seamlessly connect various communication channels, providing a cohesive and unified experience for end users across different devices.
ML-Powered Journey Mapping
When you integrate machine learning into journey mapping, it transforms how you understand and engage with customers. As a product owner, you gain knowledge into customer behaviors, preferences, and needs, allowing you to enhance customer service and raise engagement on social platforms.
ML-powered journey mapping provides a thorough health check of your customer interactions, highlighting areas for improvement.
Consider these benefits:
- Improved Customer Understanding: Gain deeper knowledge into customer behavior patterns.
- Personalized Communication: Tailor your interactions for more meaningful engagement.
- Efficient Resource Allocation: Focus efforts on areas that drive the most value.
- Enhanced Customer Satisfaction: Boost satisfaction by addressing specific needs effectively.
Personalization and User Experience
Within the area of personalization and user experience, utilizing machine learning can greatly enhance customer engagement strategies. By utilizing machine learning, you can tailor messages to millions, ensuring each user feels uniquely valued. Personalization transforms how users interact with your product, promoting loyalty and satisfaction.
You can capitalize on data-driven observations to refine user experience, delivering content that's timely and relevant. Machine learning enables dynamic modification to user preferences, elevating engagement levels.
Cross-Platform Integration Benefits
Personalization and user experience enhancements, embracing cross-platform integration can greatly enhance customer engagement strategies. By integrating systems, you streamline operations, save personal time, and boost the standard of customer service.
The deployment of 5G networks is expected to connect up to 20.4 billion Internet of Things (IoT) devices, facilitating seamless communication across platforms (Lu et al., 2020). This integration enables users to communicate seamlessly across devices, catering to diverse needs, including those with mental disabilities. By allowing users to interact with services across multiple devices, businesses can further streamline operations and enhance customer service quality.
Additionally, integrating platforms helps manage divisive subjects by ensuring consistent messaging.
Consider the following benefits:
- Increased Accessibility: Users connect from any device, ensuring broader reach and inclusivity.
- Efficient Communication: Streamlined processes reduce response times, enhancing user satisfaction.
- Unified Data Management: Centralized data helps in providing consistent service across platforms.
- Adaptability: Agile responses to evolving user needs and market trends.
Embrace these benefits to enhance your intercom system's effectiveness.
Future of Machine Learning in Intercom Systems
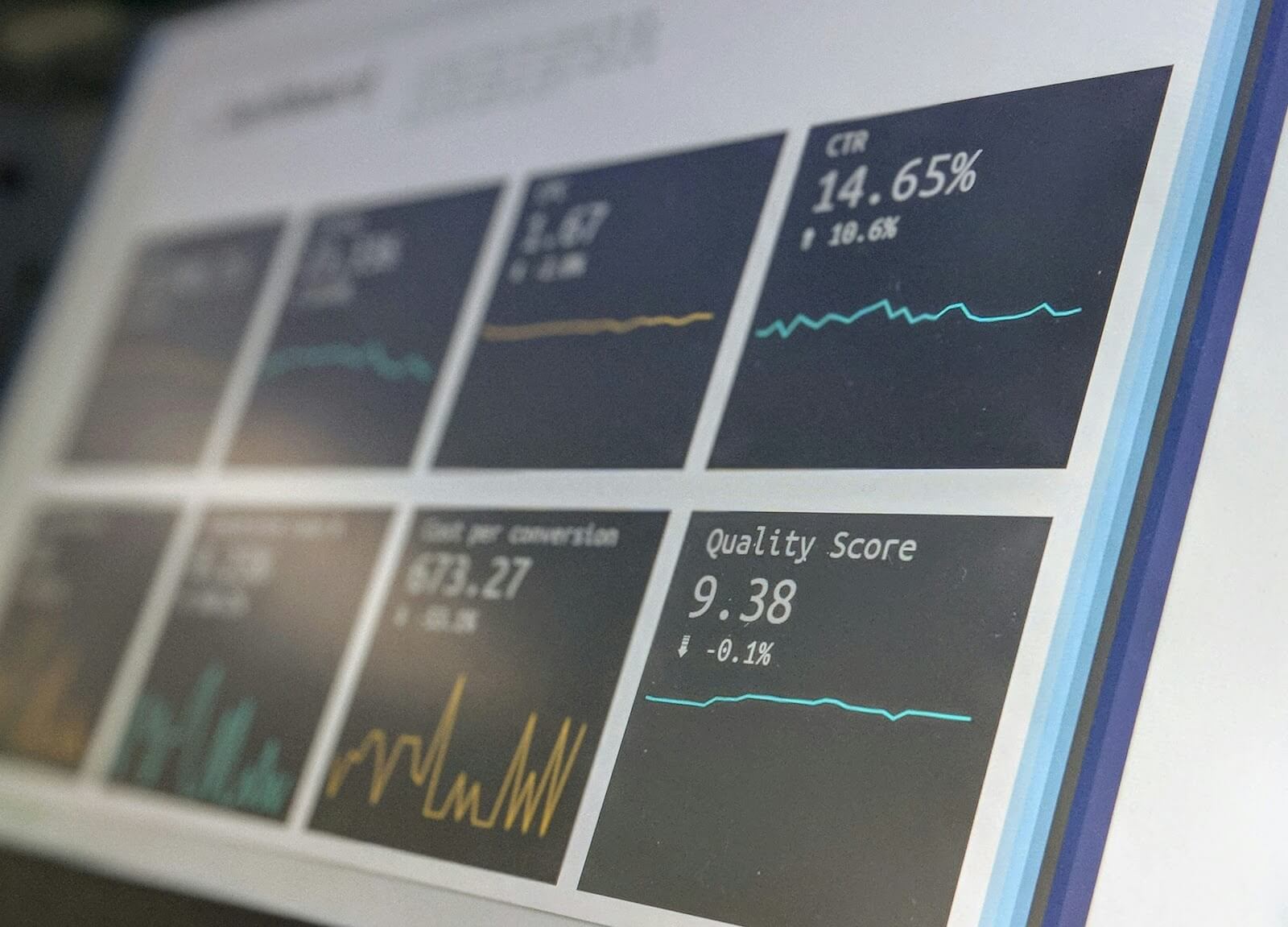
As a product owner, you can explore how emerging technologies and innovations in machine learning might transform intercom systems, enhancing user interaction and reliability. By understanding industry trends, you're positioned to identify new opportunities that can be integrated into your product, potentially offering smarter, more intuitive communication solutions. These advancements not only promise to improve the user experience but also open doors for creating features that anticipate and adjust to user needs.
Emerging Technologies and Innovations
Machine learning's rapid evolution is transforming intercom communication systems, offering product owners exciting opportunities to enhance user experiences.
As you explore emerging technologies, reflect on how machine learning is shaping software development to improve these systems. It enables better voice recognition, more accurate noise cancellation, and smarter automation, creating seamless communication experiences.
To stay ahead, focus on integrating these advancements into your development strategies.
Here are four key areas to reflect on:
- Voice Command Integration: Streamline user interaction by embedding sophisticated voice control features.
- Predictive Maintenance: Employ data-driven perspectives to anticipate and resolve issues before they impact users.
- Enhanced Security: Implement machine learning algorithms to detect and prevent unauthorized access.
- Personalized User Experiences: Tailor communication interfaces to individual preferences, increasing satisfaction.
Industry Trends and Opportunities
Innovation in intercom systems continues to thrive, driven by the dynamic field of machine learning.
Machine learning allows you to launch a beta in weeks, transforming your product into a potential cultural icon. By integrating AI-driven features, you can enhance user interaction and functionality, making your intercom system more intuitive and efficient.
The future of machine learning in intercom systems promises exciting opportunities, offering you the chance to redefine communication and stay ahead in a tech-driven market.
Why Trust Our AI and Machine Learning Insights for Intercom Systems?
At Fora Soft, we bring over 19 years of expertise in multimedia development, with a strong focus on AI-powered solutions. Our team has successfully implemented artificial intelligence features across recognition, generation, and recommendation systems, making us uniquely qualified to discuss the integration of machine learning in intercom communication systems.
Our experience in developing video streaming software and AI-powered multimedia solutions since 2005 has given us deep insights into the practical applications of machine learning in communication technologies. We've worked on projects involving WebRTC, LiveKit, and other cutting-edge technologies that are crucial for modern intercom systems. This hands-on experience allows us to provide you with not just theoretical knowledge, but practical, industry-tested insights on how machine learning can revolutionize intercom communications.
As a team that values making things "lit," we constantly push the boundaries of what's possible in AI and machine learning. Our rigorous selection process ensures that only the top 2% of candidates join our team, guaranteeing that the insights and solutions we provide are of the highest quality. With our 100% average project success rating on Upwork, you can trust that our expertise in AI and machine learning for intercom systems is backed by a track record of delivering exceptional results for our clients.
Frequently Asked Questions
How Does Machine Learning Improve Audio Quality in Intercom Systems?
You utilize machine learning to filter background noise and enhance audio clarity in intercom systems. It dynamically adjusts sound levels, ensuring clear communication by modifying to different environments, ultimately improving users' overall experience.
What Are the Hardware Requirements for ML-Powered Intercom Systems?
Your users need a strong processor, sufficient RAM, and a reliable network interface for ML-powered intercom systems. Ensure that your platform provides ample storage for data processing and supports high-quality microphones for capturing clear audio, enhancing overall system performance for your users.
Can ML Algorithms in Intercoms Adapt to New Languages?
Yes, ML algorithms in intercoms can adjust to new languages. You'd train them on diverse language datasets, ensuring they identify and process speech effectively. Regular updates help refine these capabilities, enhancing user communication experiences.
How Does Machine Learning Handle Privacy Concerns in Intercom Communications?
Algorithms can encrypt data and anonymize voice commands. By processing locally, they reduce data sharing risks, ensuring users' conversations remain private while still enhancing the system's functionality.
What Are the Common Challenges in Integrating ML Into Existing Intercom Systems?
You'll face challenges like data compatibility with existing systems, ensuring real-time processing, and maintaining user privacy. It's essential to align ML models with your system's architecture and prioritize training models using relevant, high-quality data.
To sum up
To summarize, utilizing machine learning in intercom systems transforms them into intelligent assistants, enhancing communication by personalizing user interactions and predicting needs. As a product owner, you can implement these technologies to streamline operations and boost efficiency, while considering ethical data management.
By embracing these advancements, you guarantee your intercom systems remain at the forefront of innovation, providing users with a more engaging and satisfying experience, fundamentally reshaping the future of communication in our rapidly evolving digital world.
You can find more about our experience in AI development and integration here
Interested in developing your own AI-powered project? Contact us or book a quick call
We offer a free personal consultation to discuss your project goals and vision, recommend the best technology, and prepare a custom architecture plan.
References:
Akilimalissiga, S. and Sukdeo, N. (2023). Pivotal relationship between service delivery automation and job losses: a case of the south african banking industry. https://doi.org/10.46254/af04.20230048
Lu, Y., Liu, L., Panneerselvam, J., Yuan, B., Gu, J., & Antonopoulos, N. (2020). A gru-based prediction framework for intelligent resource management at cloud data centres in the age of 5g. Ieee Transactions on Cognitive Communications and Networking, 6(2), 486-498. https://doi.org/10.1109/tccn.2019.2954388
Morrison, D., McLoughlin, I., Hogan, A., & Hayes, C. (2021). Evolutionary clustering and analysis of user behaviour in online forums. Proceedings of the International Aaai Conference on Web and Social Media, 6(1), 519-522. https://doi.org/10.1609/icwsm.v6i1.14314
Wahab, H. (2023). Exploring the effect of ai chatbots on customer experience, satisfaction and advocacy: new evidence from the banking sector in egypt. المجلة العملیة التجارة والتمویل, 43(2), 116-146. https://doi.org/10.21608/caf.2023.303703
Comments