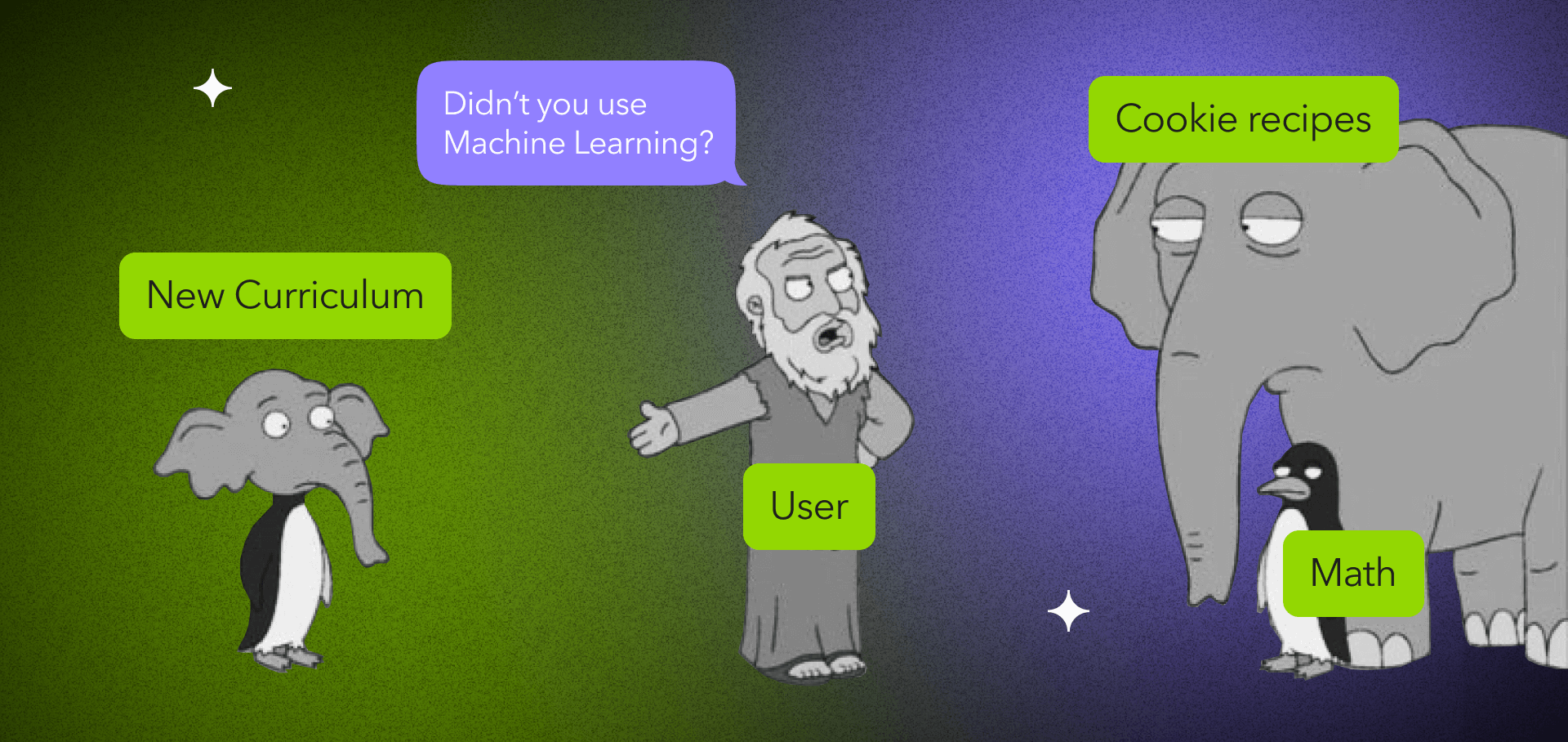
As we explore the transformative potential of machine learning in education, it's clear that this technology is revolutionizing curriculum development and learning experiences. By using the power of data analytics, advanced learning management systems (LMS) can identify individual student needs and performance gaps, enabling personalized learning experiences that keep learners engaged and motivated.
One prime example of this innovation is our BrainCert project, a customizable LMS that has redefined virtual classrooms. With its unique WebRTC-powered HTML5 Virtual Classroom, BrainCert offers an interactive whiteboard that surpasses traditional classroom experiences. This platform demonstrates how machine learning can be applied to create flexible, responsive learning environments.
The success of platforms like BrainCert underscores the significant impact of machine learning on education. As we continue to recognize the potential of this technology, we're to witness even more dramatic improvements in how we teach and learn.
Are you ready to learn the power of machine learning and its transformative impact on education?
Key Takeaways
- Machine learning analyzes student data to identify learning gaps and personalize curriculum content
- Data-driven assessments pinpoint areas of student struggle and excellence for targeted feedback and support
- AI-driven adaptive learning systems automatically adjust content and pacing to individual learner needs
- A/B testing compares curriculum variations to identify successful elements and optimize learning outcomes
- Machine learning automates grading, scheduling, and resource allocation, allowing educators to focus on high-value activities
Understanding Machine Learning In Education
To understand machine learning in education, it's important to first define what it is and how it relates to the field.
Definition And Relevance To Education
Machine learning, a subset of artificial intelligence, enables computers to learn and improve from experience without being explicitly programmed, and it's increasingly being applied in educational software to personalize learning, automate grading, and predict student performance.
By analyzing student data and interactions, machine learning algorithms can dynamically adjust the difficulty level, pace, and content presented to each learner based on their unique needs and progress. This tailored approach helps to optimize engagement, motivation, and learning outcomes. Additionally, machine learning can streamline content creation by suggesting relevant resources, generating practice questions, and providing understanding into the effectiveness of educational materials.
Identifying Learning Needs And Gaps
To identify learning needs and gaps, you can utilize data analytics to assess student performance. Machine learning algorithms can then be applied to this data to tailor curriculum content to individual students' needs.
Data Analytics For Student Performance Assessment
Data-driven student performance assessment enables educators to pinpoint learning gaps and personalize instruction for enhanced outcomes. You can utilize data analytics to gain significant understanding into student performance, identifying areas where they excel and struggle. By analyzing patterns in student outcomes, you can provide targeted feedback to students, helping them understand their strengths and weaknesses.
This data-driven approach allows you to optimize the learning process, modifying content and pacing to meet individual needs. Additionally, content generation tools powered by machine learning can assist in creating personalized learning materials tailored to each student's unique requirements.
Machine Learning For Tailoring Curriculum Content
Utilize the potential of machine learning to craft personalized curriculum content that addresses individual learning needs and bridges knowledge gaps. By analyzing student data, AI-generated content can be tailored to each learner's strengths and weaknesses.
Here are four ways machine learning enhances curriculum development:
- Responsive quizzes assess student understanding and identify areas for improvement.
- Content creation tools generate customized learning materials based on student performance data.
- Educational content creation platforms use AI to develop engaging, interactive lessons that cater to diverse learning styles.
- Machine learning algorithms continuously refine and optimize content delivery, ensuring that students receive the most effective learning experiences.
Personalizing Learning Experiences
Flexible learning systems employ predictive analytics to continuously assess learner progress and modify content accordingly. You can also use Machine Learning to generate customized assessments and targeted feedback, ensuring each user receives support tailored to their unique needs.
Adaptive Learning Systems and Predictive Analytics
Adjustable learning systems and predictive analytics revolutionize the way you personalize learning experiences for your end users.
By utilizing AI-driven understandings, you can:
- Automatically modify content and pacing to each learner's needs
- Predict areas where learners may struggle and proactively offer support
- Generate personalized content recommendations based on learning history
- Continuously optimize the learning path using real-time data
Adaptive learning systems and predictive analytics are powerful tools in modern educational technology. They enable you to create dynamic, responsive learning environments that cater to individual strengths, weaknesses, and preferences.
Customized Assessments and Feedback Mechanisms
Pairing flexible learning with customized assessments and intelligent feedback mechanisms further enhances your ability to personalize the learning journey. By utilizing AI-powered tools, you can create assessments tailored to each student's unique needs, ensuring they're challenged at the right level.
These customized assessments provide significant understanding into individual learning outcomes, allowing you to adjust course content and delivery methods accordingly. Real-time feedback, generated by sophisticated algorithms, guides students through their learning process, offering targeted suggestions for improvement and reinforcing key concepts. This immediate feedback keeps learners engaged and motivated, as they can see their progress and areas for growth. By implementing these personalized assessment and feedback systems, educational institutions can create truly responsive learning experiences that cater to the diverse needs of their students.
Data-Driven Curriculum Design
When designing your curriculum, you can utilize data to analyze the effectiveness of your approach and gauge student engagement. A/B testing allows you to compare different curriculum variations and identify the most successful elements. By making data-driven decisions, you can optimize your curriculum to maximize learning outcomes and keep students actively involved in the educational process.
Analyzing Effectiveness and Student Engagement
Utilizing machine learning algorithms, educational software can analyze student engagement and performance data to optimize curriculum design and improve learning outcomes.
AI-driven tools and platforms enable you to:
- Assess content quality and identify areas for improvement
- Personalize learning paths based on individual student needs
- Create interactive experiences that boost engagement and retention
- Monitor student progress and provide targeted support
Implementing A/B Testing For Curriculum Approaches
To further enhance your educational software's effectiveness, implement A/B testing for your curriculum approaches and apply data-driven interpretations to optimize learning outcomes. By using artificial intelligence, you can create multiple versions of educational materials and test them on different student groups. This allows you to gather actionable observations into which content variations lead to better engagement, comprehension, and knowledge retention. Based on this data, you can iteratively refine your content creation process, making targeted improvements to curriculum design.
Over time, this continuous learning loop powered by A/B testing and AI will help you zero in on the most effective pedagogical strategies for your specific student audience.
Automating Administrative Tasks
You can utilize machine learning to automate various administrative tasks in education, saving time and resources. For instance, AI-powered grading systems can quickly assess student assignments, freeing up teachers to focus on more high-value activities.
Additionally, machine learning algorithms can optimize class scheduling and efficiently allocate resources like textbooks, technology, and classroom space based on real-time data and predictive analytics.
Streamlining Grading, Scheduling, and Resource Allocation
Machine learning streamlines administrative tasks in education, automating grading, scheduling, and resource allocation to save educators time and improve efficiency.
Here are 4 ways it's transforming educational practices:
- AI-powered grading quickly assess assignments, providing immediate feedback to students.
- Intelligent scheduling tools optimize class times and locations based on availability and preferences.
- Machine Learning algorithms analyze student data to personalize learning paths and allocate resources where needed most.
- Automation handles routine admin work, freeing teachers to focus on creating effective learning experiences.
Integrating With Existing Educational Technologies
When integrating machine learning solutions with existing educational technologies, you'll need to guarantee compatibility and develop APIs. It's essential to work closely with the educational institutions' IT teams to understand their current systems and identify any potential integration challenges.
By developing sturdy APIs, you can promote seamless data exchange and enable your machine learning models to enhance the functionality of the existing educational tools.
Ensuring Compatibility and Developing APIs
Ensuring your machine learning-powered educational product seamlessly integrates with existing technologies is essential for widespread adoption and user satisfaction.
To achieve this, focus on:
- Ensuring compatibility with popular content management systems and learning platforms
- Developing strong APIs that allow your AI-powered learning platform to exchange data with other educational resources
- Thoroughly testing integrations to identify and resolve any compatibility issues
- Providing extensive documentation and support for integrating your product with existing systems
Training And Support For Educators
To effectively integrate machine learning into your educational product, you must prioritize training and support for educators.
Providing Resources and Fostering Continuous Learning
Developers must prioritize ongoing training and support for educators to guarantee successful adoption and effective use of machine learning technologies in the classroom.
Here are four key ways to provide resources and nurture continuous learning:
- Create a content creation process that involves educators in developing and refining educational videos and other digital learning content.
- Offer regular workshops and webinars to keep teachers updated on the latest machine learning tools and techniques for effective learning.
- Provide access to online forums and communities where educators can share best practices, ask questions, and collaborate with peers.
- Analyze audience preferences and feedback to tailor training materials and support resources to the specific needs and interests of educators in different subject areas and grade levels.
Monitoring And Evaluating Outcomes
To effectively monitor and evaluate the outcomes of machine learning in education, you'll need to establish clear metrics for success. These metrics should be tailored to your specific goals, whether that's improving student engagement, increasing learning retention, or streamlining administrative tasks.
Establishing Metrics and Adjusting Strategies
You'll need to establish clear metrics and continuously monitor outcomes to effectively evaluate and adjust your machine learning strategies in educational software.
Key metrics to track include:
- Engagement levels with AI-powered content delivery
- Improvements in learning outcomes and test scores
- User satisfaction ratings and feedback on the enhanced experience
- Accuracy and effectiveness of the underlying artificial intelligence algorithms
Frequently Asked Questions
How Can Machine Learning Ensure Data Privacy and Security in Educational Settings?
You can utilize machine learning to anonymize student data, implement secure access controls, and monitor for potential breaches. It's essential to prioritize data privacy and security to protect sensitive information and maintain trust in educational settings.
What Are the Potential Biases in Machine Learning Algorithms for Curriculum Development?
You should watch for biases in ML algorithms like assuming certain student demographics prefer specific subjects or learning styles. Analyze training data for skews and test outputs to guarantee fair, inclusive curriculum recommendations for all learners.
How Can Educators Without Technical Expertise Implement Machine Learning in Their Classrooms?
You can implement machine learning in your classroom by partnering with tech-savvy colleagues or seeking user-friendly AI tools. Focus on clearly defined goals, start small, and gradually expand as you gain experience and see positive results.
What Are the Costs Associated With Integrating Machine Learning in Curriculum Development?
You'll need to budget for data collection, ML software, cloud computing, and technical expertise. Costs vary but expect to invest thousands to tens of thousands for a strong solution that enhances the education curriculum's effectiveness.
How Can Machine Learning Adapt to the Evolving Needs of Learners and Educators?
You can utilize machine learning's flexibility to personalize learning paths, analyze student data in real-time, and continuously optimize content delivery based on individual progress and feedback, ensuring the curriculum stays relevant to learners' and educators' evolving needs.
To sum up
You've seen how machine learning revolutionizes education, personalizing learning, automating tasks, and driving data-driven curriculum design. By identifying gaps, tailoring content, and providing real-time feedback, it enhances student engagement and outcomes.
Integration with existing technologies and educator training guarantee successful implementation. Through continuous monitoring and evaluation, machine learning refined approaches, optimizing learning experiences. Utilize this transformative technology to release students' full potential, enable educators, and shape the future of education.
Interested in developing your own AI-powered project? Contact us or book a quick call
We offer a free personal consultation to discuss your project goals and vision, recommend the best technology, and prepare a custom architecture plan.
You can find more about our experience in AI development and integration here
For further insights into our AI-centric approach to educational app development, explore the interview with ALDA CEO
Comments