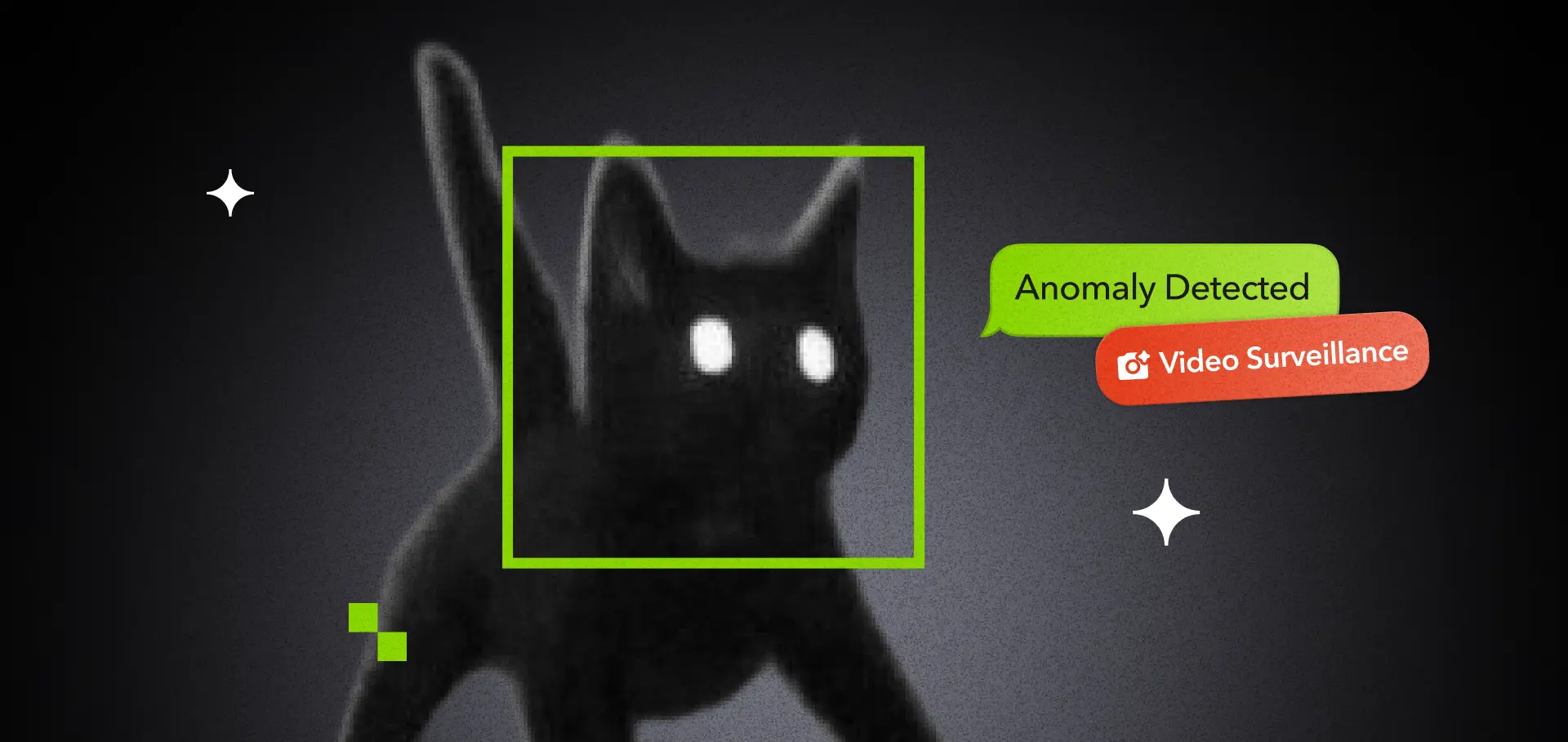
Imagine walking into a security control room in 2025, where smart cameras don't just record - they think. Modern AI video systems are changing how we keep spaces safe, spotting odd behavior faster than human eyes and saving money along the way. Whether protecting a shop, factory, or transport hub, getting to know these clever systems will help stay ahead of security threats.
Key Takeaways
- Advanced features combine object recognition, behavioral analysis, and predictive alerts for comprehensive real-time threat detection.
- Implementation across retail, manufacturing, and transportation sectors enables the detection of specific anomalies like theft and safety violations.
- Edge computing and cloud solutions offer different trade-offs between latency, privacy, and scalability for video processing requirements.
- Privacy-preserving techniques, encryption protocols, and data retention policies ensure compliance while maintaining system effectiveness.
💡 Ready to revolutionize your video surveillance system?
Our team has been implementing AI-powered solutions since 2005. Check out our portfolio or schedule a free consultation to discuss your specific needs.
Understanding AI Video Surveillance
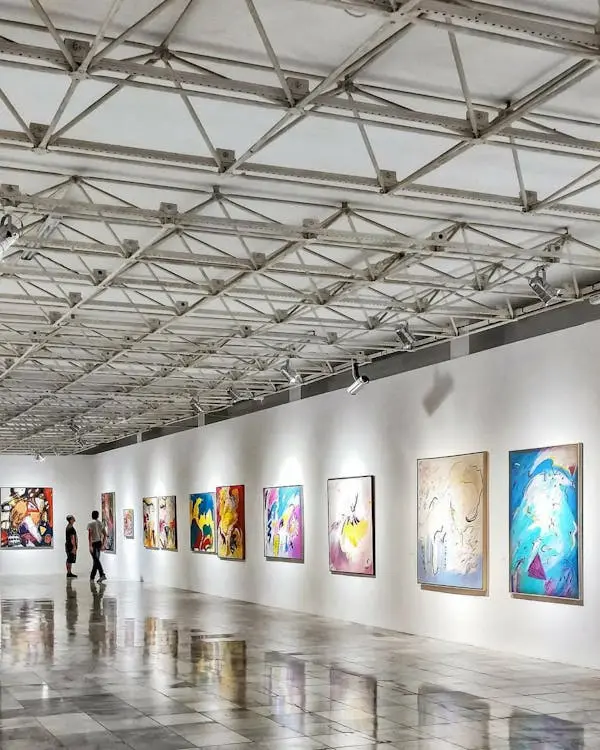
Organizations can now detect security threats in real-time as AI-powered surveillance systems continuously analyze video feeds and identify unusual patterns without human fatigue. This capability is particularly crucial for maintaining consistent vigilance over extended monitoring periods (Ahmed & Echi, 2021).
Modern AI surveillance platforms let you implement advanced features like object recognition, behavioral analysis, and predictive alerts that weren't possible with traditional CCTV monitoring.
Your development team can enhance these capabilities by integrating computer vision APIs and machine learning models that improve detection accuracy over time through automated training on your specific security scenarios.
Why Organizations Are Rapidly Adopting AI Surveillance
While traditional video surveillance has long been a cornerstone of security systems, organizations are shifting towards AI-powered solutions to address increasingly complex security challenges.
AI-driven security features are revolutionizing how security teams process and analyze vast amounts of video data. Advanced video analytics enable real-time monitoring of multiple feeds simultaneously, helping detect security threats more efficiently than human operators alone.
AI-powered anomaly detection systems can identify and respond to security incidents four times faster than traditional systems, significantly improving response times (Satyasree, 2023).
Through continuous learning and pattern recognition, these systems provide actionable information that helps your team make data-driven decisions. This automation reduces human error and allows your security personnel to focus on critical incidents requiring immediate attention.
How AI Transforms Traditional Video Monitoring
Your AI video surveillance system can deliver measurable returns through reduced security staffing costs while increasing detection accuracy compared to human monitoring alone.
By implementing AI-powered anomaly detection, you'll identify and respond to security incidents an average of four times faster than traditional systems, leading to significant reductions in loss prevention costs. Recent research has validated this enhanced response capability, demonstrating that AI-powered systems consistently achieve faster incident detection and response compared to conventional approaches (Mızrak, 2024).
The integration of AI analytics also provides actionable business intelligence through features like crowd density monitoring, object tracking, and behavioral pattern analysis - helping you make data-driven decisions about facility operations and security protocols.
Key Benefits and ROI Statistics
Three key financial advantages drive the adoption of AI-powered video surveillance systems in modern businesses. AI-powered features and advanced video analytics capabilities reduce manual monitoring costs. Proactive video monitoring enhances operational efficiency through automated threat detection. Your business can achieve substantial cost savings by minimizing false alarms and optimizing security staff deployment.
Implementing AI-Powered Anomaly Detection
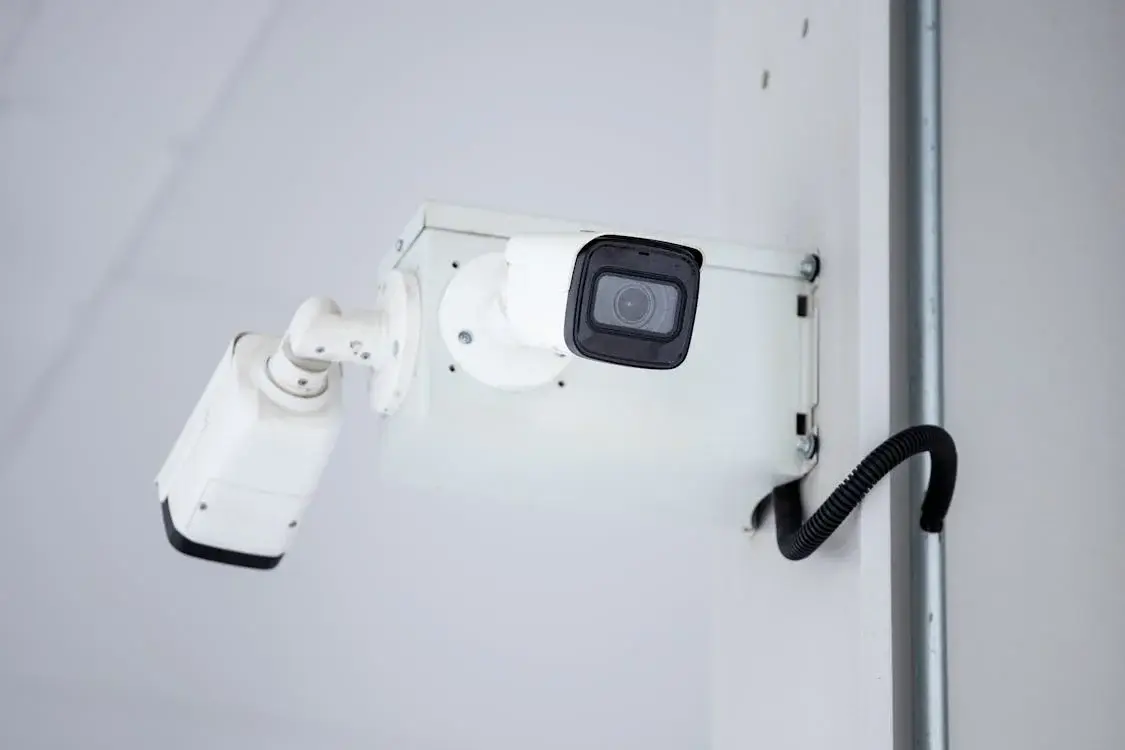
Your AI-powered video surveillance system needs two main components: a trained machine-learning model to analyze video feeds and a strong data pipeline to process incoming footage in real time. The model can detect various types of anomalies including unauthorized access, suspicious behavior patterns, and objects left in restricted areas. You'll want to implement both supervised learning for known threat detection and unsupervised learning algorithms to identify new, previously unseen anomalous activities in your surveillance footage.
Core Components of AI Video Analytics
Building AI-powered video analytics requires several interconnected components that form the foundation of an effective anomaly detection system. Your advanced video surveillance solutions need to integrate strong video management software that controls data ingestion and storage. The artificial intelligence layer processes this data through specialized algorithms for motion detection and pattern recognition. You'll need to implement video analytics modules that handle real-time processing, event classification, and alert generation. These components must work seamlessly together, transforming raw video feeds into actionable understandings while maintaining system performance and reliability.
Building Enterprise-Grade Surveillance Solutions: The V.A.L.T Journey
When developing V.A.L.T, our team focused on creating a surveillance system that could scale without compromising on security or usability. The development process revealed key insights about enterprise surveillance needs:
- Modularity is crucial - The system must adapt to different use cases while maintaining simplicity
- Security cannot be an afterthought - We implemented enterprise-grade encryption and granular permission controls
- Automation saves resources - Features like scheduled recording and automated PTZ adjustments reduce manual oversight
- Integration flexibility matters - Supporting industry-standard cameras and protocols ensures long-term viability
This experience shaped our understanding of how AI-powered surveillance systems should balance complexity with user-friendliness.
🎯 Want to see AI video analytics in action?
We've successfully implemented these solutions across multiple industries. Explore our AI integration services or chat with our experts to learn how we can enhance your surveillance systems.
Types of Anomalies AI Can Detect
Your AI-powered surveillance system can directly enhance security across diverse sectors including retail, manufacturing, healthcare, and transportation. In retail environments, you'll be able to detect suspicious behaviors like shoplifting or unusual customer movements.In manufacturing settings, the system can monitor for safety violations or equipment malfunctions. Transportation hubs can benefit from your AI implementation by identifying abandoned objects, unauthorized access attempts, or crowd behavior anomalies that might indicate security threats.
Real-World Applications Across Industries
Modern surveillance systems equipped with AI can detect a wide range of anomalies across different industries, from retail security to industrial safety monitoring. Studies have shown that AI integration can enhance threat detection capabilities by up to 90% in both retail and industrial applications (Nadaf et al., 2023). AI-enabled mobile and fixed video solutions deliver advanced security understanding in real-time, while pattern-based video analytics enhance your surveillance capabilities. These video monitoring technologies offer thorough security coverage through automated threat detection and instant alerts.
Case Study: V.A.L.T - Advanced Video Surveillance in Action
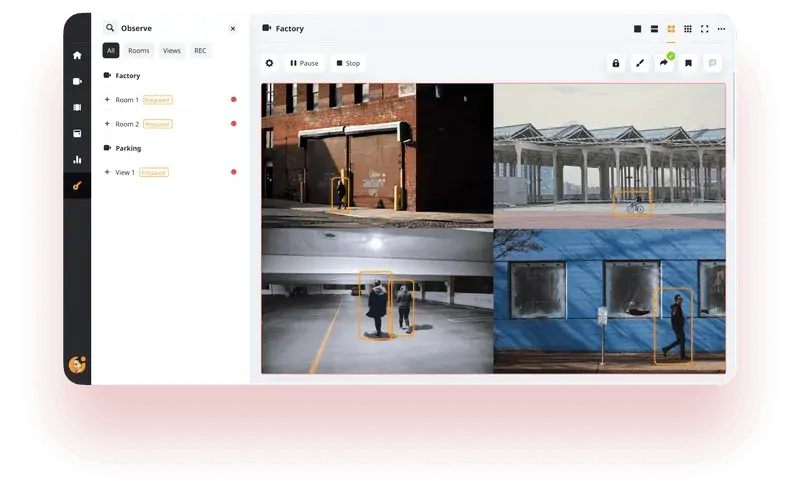
Our V.A.L.T system demonstrates the practical implementation of AI-powered surveillance across diverse sectors. Currently serving over 450 organizations, including police departments, medical institutions, and child advocacy centers, V.A.L.T showcases how intelligent video surveillance can be both powerful and user-friendly. The system supports HD video streaming from multiple IP cameras with advanced PTZ controls, while maintaining strict security protocols through SSL and RTMPS encryption. For instance, in medical education settings, the system enables precise supervision of patient consultations while ensuring strict access controls - students can only access their own consultation recordings, protecting patient privacy.
Maximizing AI Surveillance Effectiveness
Your choice of AI implementation method will directly impact your video surveillance system's effectiveness, with options ranging from pre-trained models to custom solutions tailored to your specific use case. To successfully implement AI surveillance, you'll need strong computing infrastructure, high-quality video feeds, and sufficient data storage capacity for training and operation.
Your development team should also consider scalability requirements, real-time processing capabilities, and integration protocols that align with your existing security infrastructure.
Choosing the Right AI Implementation Method
Your video surveillance system's effectiveness will largely depend on whether you choose cloud-based or edge-computing implementation for AI processing.
Cloud solutions offer scalable computing capability and centralized data storage, but they require stable internet connectivity and may introduce latency in real-time detection scenarios.
Edge computing brings AI processing directly to your surveillance cameras, enabling faster response times and better privacy controls, though you'll need to carefully consider hardware requirements and processing limitations.
Cloud vs. Edge Computing Solutions
When implementing AI surveillance systems, deciding between cloud and edge computing architectures directly impacts performance, costs, and scalability. Cloud computing solutions offer unlimited storage and advanced analytics capabilities, but may introduce latency.
Edge computing solutions process data locally, enabling real-time monitoring and faster response times in your video surveillance systems, though with more limited computational resources. Recent research has shown that edge computing significantly enhances video surveillance systems through local data processing, leading to improved response times (Chen et al., 2022).
⚡ Not sure whether to choose cloud or edge computing for your AI surveillance system?
Let our experts help you make the right choice. Book a 30-minute strategy session - we'll analyze your requirements and recommend the optimal solution.
Essential Technical Requirements
Your AI surveillance system needs to seamlessly connect with your current video management software (VMS) and security infrastructure through standardized APIs and protocols. You'll want to guarantee your chosen AI solution supports common video formats, and compression standards, and can process feeds from different camera manufacturers without compatibility issues. Integration planning should include considerations for database management, user authentication systems, and alert mechanisms that work with your existing security workflows.
Integration with Existing Systems
Successful implementation of AI surveillance systems requires seamless integration with existing security infrastructure and databases. Your video surveillance solutions must work harmoniously with current video management systems while addressing modern security challenges through advanced technologies. This integration hinges on several key factors, including:
- Guarantee API compatibility with legacy systems
- Implement standardized data exchange protocols
- Configure automated alert synchronization
- Establish secure communication channels
- Deploy unified control interfaces for operators
Future-Proofing Your AI Surveillance Strategy
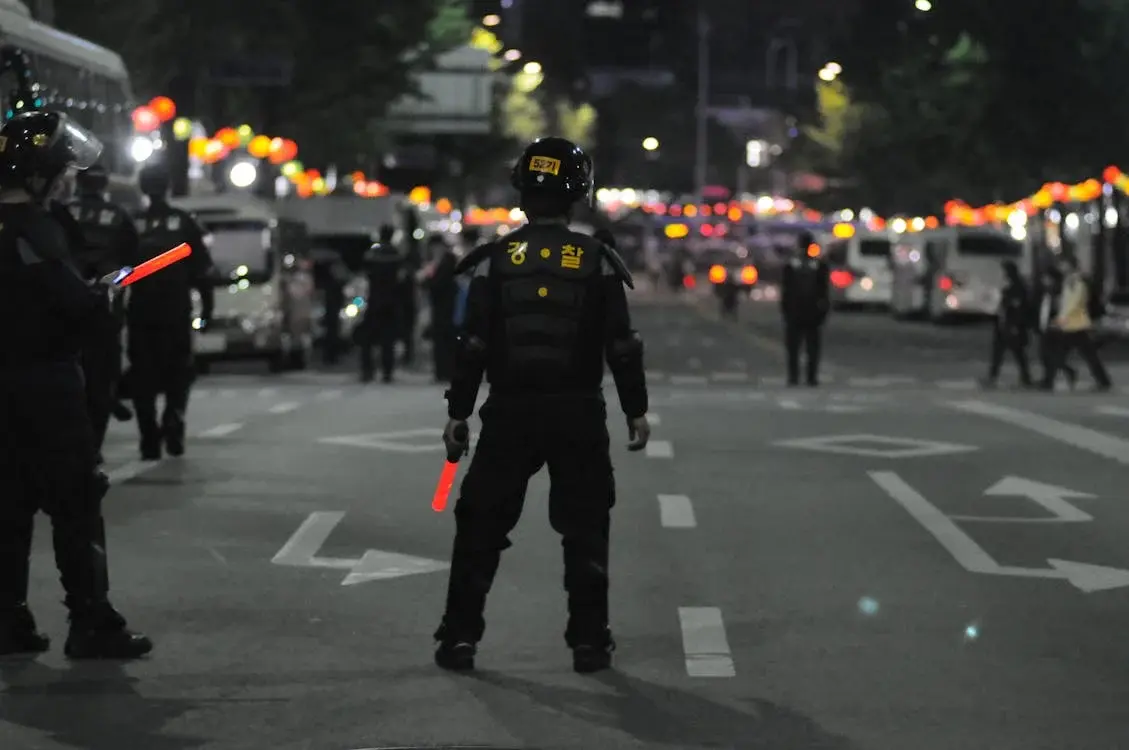
Your AI surveillance system's long-term success depends on staying current with emerging technologies like federated learning and edge computing that can enhance detection capabilities while reducing latency. You'll need to implement strong data governance frameworks and privacy-preserving techniques to maintain compliance with evolving regulations around AI surveillance. Consider adopting modular architecture patterns that allow you to easily integrate new AI models and features as they become available, ensuring your system can adjust to changing security requirements.
Emerging AI Surveillance Technologies
While AI surveillance technologies continue to evolve rapidly, staying ahead of emerging trends has become critical for product development success. Advanced surveillance cameras now integrate with self-learning video analytics to provide more accurate threat detection. Video surveillance experts recommend implementing flexible advanced video solutions that can adjust to new capabilities as they emerge. To ensure your surveillance products remain cutting-edge and adaptable, consider the following key strategies:
- Implement modular AI architectures to easily integrate future technologies
- Add support for edge computing to enhance real-time processing
- Design scalable storage solutions for increasing video data demands
- Build APIs that enable integration with emerging AI surveillance technologies
- Develop flexible alert systems that can incorporate new detection parameters
Privacy and Compliance Considerations
To future-proof your AI surveillance system's data protection, you'll need to implement strong encryption protocols and access controls at both the application and database levels. You should establish clear data retention policies that automatically purge surveillance footage after defined periods while maintaining detailed audit logs of all system interactions. Your development roadmap must include regular security assessments and updates to comply with evolving privacy regulations like GDPR and CCPA, incorporating privacy-by-design principles into each feature release.
Best Practices for Data Protection
Protecting user data in AI surveillance systems requires an exhaustive strategy that addresses both current privacy regulations and anticipated future compliance requirements. When implementing data protection measures, you'll need custom solutions that integrate advanced encryption technology to safeguard your video evidence and surveillance equipment. These solutions should include the following:
- Implement end-to-end encryption for all video streams
- Deploy multi-factor authentication for system access
- Establish automated data retention policies
- Create detailed audit trails for all data interactions
- Set up secure backup protocols with encryption at rest
Why Trust Our AI Surveillance Expertise?
We've been at the forefront of video surveillance innovation since 2005, successfully implementing AI-powered solutions for over 450 organizations worldwide. Our team's deep expertise in multimedia development and artificial intelligence has led to groundbreaking implementations across various sectors, from law enforcement to healthcare. With a proven track record of 100% project success rating and less than 1% of clients rating their results as not totally successful, we understand the intricate balance between advanced AI capabilities and practical implementation needs.
Our expertise spans the complete spectrum of AI video surveillance - from developing custom recognition algorithms to implementing enterprise-grade security protocols. We've helped organizations navigate complex technical challenges, including real-time processing requirements, multi-camera synchronization, and seamless integration with existing infrastructure. This hands-on experience, combined with our rigorous developer selection process (where only 1 in 50 candidates receives an offer), ensures that our insights come from real-world implementation success rather than theoretical knowledge.
Frequently Asked Questions
How Can Developers Integrate Legacy Camera Systems With New AI Surveillance Platforms?
You can integrate legacy cameras by developing REST API connectors, using RTSP protocol bridges, implementing video stream converters, and creating middleware that normalizes different video formats for AI processing compatibility.
What Programming Frameworks Are Recommended for Custom AI Surveillance Model Development?
TensorFlow and PyTorch are ideal for custom surveillance models. Consider using OpenCV for image processing, and YOLO or Detectron2 frameworks when you need pre-trained models for object detection.
How Do You Handle Real-Time Data Streaming Bottlenecks in AI Video Processing?
You'll reduce streaming bottlenecks by implementing data buffering, using GPU acceleration, optimizing frame rates, and applying parallel processing pipelines. Consider using message queues like Kafka for efficient data handling.
Which APIS Best Support Multi-Camera Synchronization for Distributed Surveillance Systems?
You'll get reliable multi-camera sync using ONVIF's API for standardized integration, RTSP for streaming, or GStreamer's API for pipeline control. AWS Kinesis and Azure Media Services offer scalable cloud-based synchronization solutions.
What Are the Computational Requirements for Edge Processing Versus Cloud-Based Detection?
You'll need 2-4GB RAM and quad-core processors for basic edge detection, while cloud processing demands 8-16GB RAM per stream. Edge requires less bandwidth but has limited processing capability versus scalable cloud resources.
To Sum Up
As you prepare for 2025's AI surveillance landscape, you'll need to balance state-of-the-art detection capabilities with practical implementation. Focus on scalable solutions that integrate seamlessly with existing systems while maintaining high accuracy and minimal false positives. By staying current with AI frameworks, investing in strong testing protocols, and prioritizing user-friendly interfaces, you'll position your surveillance products to meet evolving security demands effectively.
🚀 Ready to future-proof your surveillance system?
With 19+ years of experience in multimedia development and a 100% project success rate, we're here to help you implement cutting-edge AI surveillance solutions. View our track record or schedule a call to start your AI transformation journey today.
References:
Ahmed, A., & Echi, M. (2021). Hawk-eye: An AI-powered threat detector for intelligent surveillance cameras. IEEE Access, 9, pp. 63283-63293. https://doi.org/10.1109/access.2021.3074319
Chen, Y., Lin, Y., Hu, Y., et al. (2022). Distributed real-time object detection based on edge-cloud collaboration for smart video surveillance applications. IEEE Access, 10, pp. 93745-93759. https://doi.org/10.1109/access.2022.3203053
Mızrak, F. (2024). Integrating AI with aviation cybersecurity: The strategic role of human resources. Business and Management Studies: An International Journal, 12(3), pp. 610-628. https://doi.org/10.15295/bmij.v12i3.2396
Nadaf, J., Patil, T. B., Lavate, R. K., et al. (2023). Innovative AI-driven automation system leveraging advanced perceptive technologies to establish an ideal self-regulating video surveillance model. Tuijin Jishu/Journal of Propulsion Technology, 44(2). https://doi.org/10.52783/tjjpt.v44.i2.220
Satyasree, E. (2023). Edge AI for real-time video analytics in surveillance systems. International Journal on Recent and Innovation Trends in Computing and Communication, 11(10), pp. 2269-2275. https://doi.org/10.17762/ijritcc.v11i10.8947
Comments