
AI-powered IP cameras are changing how we think about security cameras - they're like traditional cameras that went to tech school. Instead of just recording footage, these smart systems can spot objects, analyze behavior, and even recognize faces in real-time. Whether processing information through cloud systems or right on the camera itself, these AI cameras turn simple video feeds into useful security insights.And this is just the beginning - new features keep rolling out as the technology grows smarter.
Key Takeaways
- AI-powered cameras integrate advanced facial recognition and behavioral analysis, transforming raw footage into actionable intelligence for enhanced security monitoring.
- Edge computing capabilities enable local data processing, reducing latency and bandwidth usage while improving real-time threat detection.
- Machine learning algorithms establish baseline activity patterns and automatically flag anomalies, leading to more accurate security alerts.
- Integration of 5G networks and cloud processing enables scalable solutions with improved video quality and faster data transmission.
- Privacy-focused features like automatic face blurring and end-to-end encryption ensure compliance with data protection regulations while maintaining security.
💡 Ready to enhance your surveillance system with AI capabilities?
Our team has 19+ years of experience in multimedia development and AI integration. Check out our past projects or schedule a free 30-minute consultation to discuss your requirements.
The Evolution of IP Camera Technology

Your traditional IP surveillance system's core functions of recording and streaming footage can be transformed by integrating AI capabilities that enable advanced features like real-time object detection and behavioral analysis. AI-enhanced systems can provide real-time insights, enabling organizations to mitigate risks and vulnerabilities more effectively than traditional security measures (Kumar, 2023).
The most notable difference between conventional and AI-enhanced systems in their ability to process data locally, reducing bandwidth usage while improving response times for critical security events.This is particularly valuable in environments with limited connectivity (Satyasree, 2023).
When choosing between these options, you'll need to weigh the enhanced capabilities of AI systems against their current limitations, such as higher computational requirements and the need for regular algorithm updates to patch security vulnerabilities.
Traditional IP Cameras vs. AI-Enhanced Systems
While traditional IP cameras have served as the foundation of video surveillance for over two decades, the integration of artificial intelligence has revolutionized their capabilities and applications.
AI-powered video surveillance systems offer advanced features that traditional security cameras can't match. Modern video analytics technology enables real-time monitoring with intelligent object detection, facial recognition, and behavioral analysis. AI systems integrated with IP cameras improve operational efficiency by automating routine monitoring tasks, allowing security personnel to focus on critical decision-making processes (Ong, 2023).
Your advanced surveillance systems can now automatically identify suspicious activities, track movement patterns, and generate instant alerts.
When upgrading your infrastructure, you'll gain access to automated reporting, predictive analytics, and enhanced search capabilities that transform raw footage into actionable observations.
Current Limitations and Security Gaps
Your IP surveillance system's effectiveness can be limited by false alarms triggered by motion detection algorithms that don't properly distinguish between relevant threats and harmless movements like swaying trees or passing animals.
While real-time video analysis capabilities have improved considerably, processing constraints still affect how quickly your system can identify and respond to genuine security events, especially when handling multiple high-resolution camera feeds simultaneously.
You'll need to contemplate implementing AI-powered filtering systems and edge computing solutions to reduce these limitations and improve the accuracy of threat detection while maintaining efficient processing speeds.
🔍 Struggling with false alarms and processing constraints?
Let our AI experts help you optimize your surveillance system. Learn about our AI integration services or contact us for a quick chat about your challenges.
False Alarm Challenges
Despite significant advances in AI-powered surveillance, false alarms remain a persistent challenge for IP camera systems, consuming precious resources and potentially undermining user confidence.
The application of machine learning techniques has shown promising results in enhancing the accuracy of anomaly detection systems, significantly reducing false alarm rates in surveillance applications (Gadal et al., 2022).
You'll need to optimize your anomaly detection algorithms to reduce false positives during real-time analysis. Consider implementing multi-layered verification protocols and fine-tuning your AI models to align with specific video surveillance trends.
Real-Time Analysis Constraints
Modern IP camera systems face considerable processing constraints when conducting real-time video analysis, particularly as resolution quality and data streams continue to increase. To optimize your AI-driven video analytics, you'll need to balance deep learning capabilities with available computing resources. Consider implementing edge computing solutions to reduce latency for real-time alerts and maintain reliable remote monitoring while delivering actionable information.
Revolutionary AI Features Transforming Surveillance
Integrating AI-powered threat detection into your surveillance system can dramatically improve security through automated recognition of suspicious behaviors, objects, and patterns.
Machine learning algorithms let you establish baseline activity patterns and instantly flag anomalies, giving your team more time to focus on real threats rather than constant manual monitoring.
Advanced Threat Detection Capabilities
To enhance your surveillance system's threat detection capabilities, you'll want to implement AI-powered facial recognition and biometric analysis that can identify and track individuals with high accuracy across multiple camera feeds.
You can strengthen security by adding behavioral pattern recognition algorithms that learn normal activity patterns and flag unusual movements or actions that may indicate potential threats. These AI features work together to create a proactive security system that spots suspicious activities before they escalate into serious incidents.
Facial Recognition and Biometric Analysis
Facial recognition and biometric analysis stand at the forefront of AI-powered surveillance development, offering product owners unprecedented capabilities for user authentication and security enhancement. You can integrate real-time threat detection by implementing facial recognition technology alongside advanced biometric analysis features. When developing these systems, make certain you're addressing privacy concerns through strong data protection protocols and user consent mechanisms.
Behavioral Pattern Recognition
While traditional surveillance systems rely on basic motion detection, modern behavioral pattern recognition employs advanced AI algorithms to analyze and interpret complex human activities in real time. Through deep learning and intelligent video analytics, you'll enhance your system's object recognition capabilities. AI-powered video analytics enable intricate behavior analysis to detect suspicious patterns, unauthorized access, and potential security threats automatically.
Predictive Analytics and Machine Learning
Your software's predictive analytics can utilize historical data patterns to anticipate potential security threats before they materialize. You'll transform your surveillance system from reactive to proactive by implementing machine learning algorithms that continuously learn from past incidents and user behaviors. These AI-driven observations enable you to develop automated response protocols and customize security measures based on your specific threat landscape.
⚡ Want to implement these cutting-edge AI features in your surveillance system?
Our team specializes in AI recognition, generation, and recommendations. Book a call to explore how we can transform your security infrastructure.
Historical Data Analysis
Modern surveillance systems employ the strength of historical data analysis to reveal patterns and trends that were previously impossible to detect. By implementing advanced analytics within your cloud-based video surveillance platform, you'll access real-time observations from archived footage. The video surveillance industry now enables you to identify recurring patterns, analyze user behavior, and make data-driven decisions for enhanced security measures.
Proactive Security Measures
Through advanced AI-powered predictive analytics, surveillance systems now anticipate potential security threats before they materialize. You'll gain enhanced situational awareness with advanced features that analyze behavior patterns in real-time, allowing your security team to respond proactively rather than reactively.
This enhanced awareness is achieved through several key capabilities, including:
- AI-powered cameras detect unusual activities and movement patterns
- Threat detection algorithms identify potential security risks instantly
- Real-time alerts notify security personnel of suspicious behavior
- Automated response protocols trigger based on predefined security scenarios
Technical Infrastructure for AI-Powered Cameras
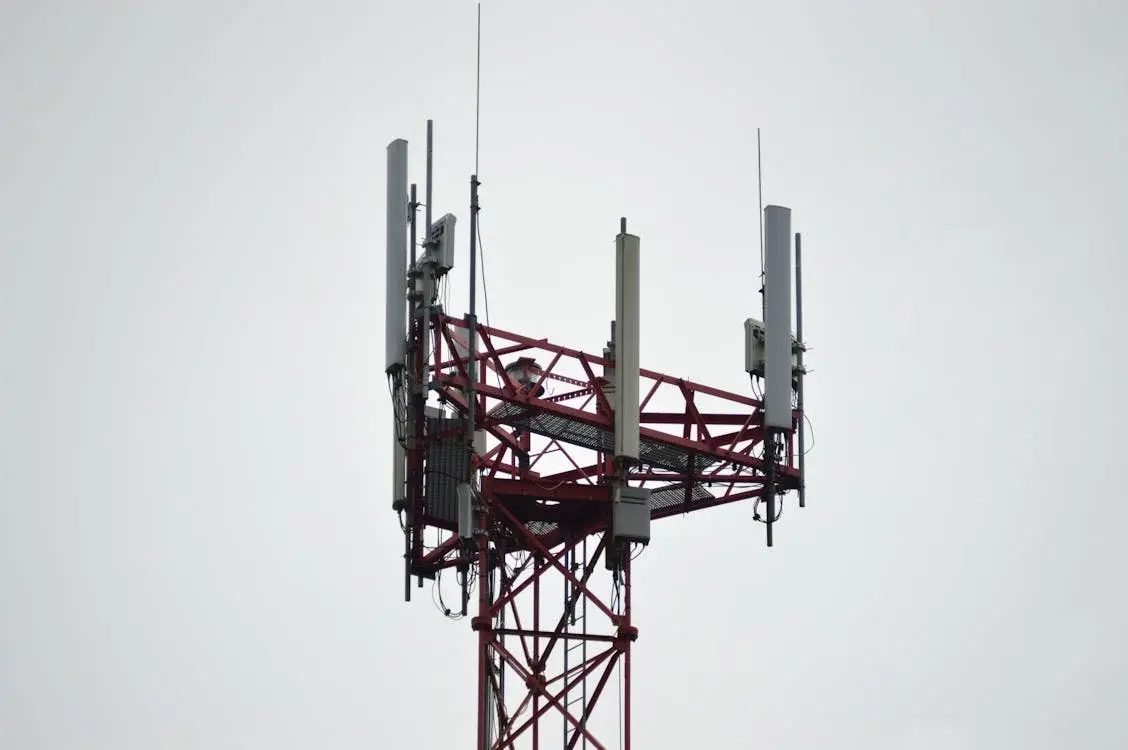
When planning your AI surveillance system's infrastructure, you'll need to evaluate whether 5G and edge computing capabilities can enhance your real-time processing needs.
You can process AI analytics either through cloud servers or directly on edge devices, with each approach offering distinct advantages for latency, bandwidth usage, and scalability.
Your choice between cloud and edge processing will depend on factors like your camera locations, network reliability, and specific AI feature requirements such as facial recognition or object detection speed.
5G Integration and Edge Computing Benefits
Edge computing brings real-time processing directly to your surveillance system by handling AI computations at or near the camera location, rather than sending all data to distant servers.
You'll see considerable reductions in latency when deploying AI features like facial recognition and motion detection, as processing happens locally within milliseconds instead of requiring round trips to the cloud.
Your system's reliability improves greatly since cameras can continue basic AI functions even during network interruptions, while also reducing your bandwidth usage and associated costs. By processing data locally, edge computing can reduce bandwidth consumption by up to 70%, as only relevant data needs to be transmitted to the cloud (Ke et al., 2021).
Real-Time Processing Capabilities
Modern AI-powered surveillance systems rely heavily on strong real-time processing capabilities to deliver instant understanding and responses. Your edge analytics and cloud-based video surveillance system work together to process data efficiently, enabling faster decision-making and immediate threat detection.
To achieve this, consider the following key steps:
- Implement distributed processing across multiple edge devices
- Configure automatic failover mechanisms for uninterrupted monitoring
- Optimize bandwidth usage through selective cloud data transmission
- Set up real-time alerts and automated response workflows
For instance, in our V.A.L.T system implementation, we achieved high-quality HD video streaming from 9 IP cameras simultaneously on a single screen, maintaining perfect AV sync while enabling real-time pan-tilt-zoom functionality. This demonstrates how proper infrastructure planning can support complex multi-camera operations without compromising performance.
Reduced Latency Solutions
Successful AI-powered surveillance implementations demand ultra-low latency solutions to process and analyze video feeds in near real-time. You can achieve reduced latency by implementing edge computing on your smart security cameras, which process data locally instead of relying solely on cloud-based solutions.
This approach optimizes real-time monitoring by minimizing network delays and bandwidth constraints.
Cloud vs. Edge Processing Considerations
When deciding between cloud and edge processing for your AI surveillance system, you'll need to evaluate your scaling needs against your network capabilities and latency requirements.
You can optimize data management by implementing hybrid processing protocols that distribute computational loads between local edge devices and cloud servers based on real-time demands.
Your system's architecture should account for bandwidth limitations while maintaining quick response times, which often means processing critical data locally while utilizing the cloud for deeper analytics and storage.
Scalability Options
Selecting the right processing infrastructure for AI-powered surveillance systems demands careful consideration of both cloud and edge computing options. Smart cameras integrated with cloud platforms offer flexible scalability options and remote management capabilities for your advanced security solutions.
To further enhance your security infrastructure, consider these additional strategies:
- Deploy containerized AI models for easy updates and maintenance
- Implement load balancing across multiple edge devices
- Employ auto-scaling features in cloud services
- Set up distributed processing nodes for regional coverage
Data Management Protocols
Effective data management protocols form the foundation of any AI-powered surveillance infrastructure, requiring careful consideration of both cloud and edge processing capabilities. When implementing video surveillance systems with artificial intelligence, you'll need to establish clear protocols for data handling, storage, and processing. Machine learning models can help automate security technologies while maintaining data integrity through structured management frameworks.
Implementation Challenges and Solutions
To guarantee your AI surveillance system's success, you'll need strong privacy and data protection protocols that comply with relevant regulations while preserving system functionality. Your development team should implement end-to-end encryption, secure user authentication, and data anonymization features in the early stages of system design.
Integration requirements demand careful attention to API compatibility, scalable architecture design, and seamless communication between your existing security infrastructure and new AI components.
When developing V.A.L.T, we addressed these challenges through:
- Browser-based point-and-click interface requiring no installation
- Modular interface design showing only relevant features to specific user roles
- Hardware controls integration for physical recording management
Privacy and Data Protection Protocols
While implementing AI surveillance systems demands strong security measures, the integration of privacy and data protection protocols presents unique challenges for product owners.
You'll need to verify your facial recognition systems comply with data protection laws while maintaining functionality. Advanced technology requires implementing sturdy privacy protections to address security concerns.
To achieve this, consider the following key measures:
- Implement end-to-end encryption for all video streams and stored data
- Design user authentication with role-based access control and audit logging
- Configure automated data retention policies that comply with regional regulations
- Develop privacy-preserving features like automatic face blurring and data anonymization
Our experience with V.A.L.T's implementation in medical education and police departments has shown the importance of granular permission controls. For example, we implemented strict access protocols ensuring medical students could only access their own patient consultation recordings, while supervisors had limited access to only their assigned students' footage.
System Integration Requirements
When integrating AI surveillance systems into your existing infrastructure, you'll need to establish strong API connections that enable seamless data flow between your new AI components and current security platforms.
Your development team should prioritize building standardized REST APIs or GraphQL interfaces that can handle real-time video streams while maintaining compatibility with legacy systems through appropriate middleware solutions.
To guarantee successful integration, you'll want to implement thorough API documentation and version control practices while considering scalability requirements for future system expansions.
API Integration Strategies
Successful implementation of AI surveillance systems depends heavily on strong API integration strategies that connect various system components. When developing for the video surveillance market, you'll need to guarantee seamless data flow between AI-driven analytics and security camera technology through centralized management platforms.
To achieve this, consider the following best practices:
- Design REST APIs with standardized authentication protocols
- Implement sturdy error handling and data validation mechanisms
- Create detailed API documentation for third-party integrations
- Structure endpoints to support real-time video processing requirements
Legacy System Compatibility
Building upon strong API strategies, legacy system compatibility presents distinct technical hurdles in AI surveillance implementation. You'll need to guarantee your existing video feeds integrate smoothly with new AI capabilities.
Consider forming strategic partnerships with vendors who specialize in bridging older security systems with modern solutions, enabling enhanced safety features while preserving your current infrastructure investment.
Real-World Applications
Real-world applications of AI surveillance can improve your product's value across multiple industries, from retail analytics tracking customer behavior to manufacturing quality control systems that detect defects in real time.
You'll find measurable ROI through reduced operational costs, with successful implementations reporting efficiency gains in the first year of deployment.
Your development team can integrate these capabilities through flexible APIs and pre-trained models, allowing for rapid prototyping and scalable solutions that adjust to your specific business requirements.
Industry-Specific Use Cases
In your retail security development roadmap, you'll want to contemplate integrating facial recognition modules that can detect known shoplifters and analyze customer traffic patterns to optimize your store layout.
Your industrial monitoring systems can benefit from machine learning algorithms that predict equipment failures and automate quality control processes in real time.
These AI-powered solutions offer you scalable options to enhance both security and operational efficiency through features like anomaly detection, automated alert systems, and predictive maintenance scheduling.
Retail Security Solutions
Modern retail environments have transformed their security approaches through AI-powered surveillance solutions that protect assets while enhancing the customer experience.
You'll benefit from security camera systems that analyze video footage in real time, detect unusual activities, and provide remote access to multiple locations.
To further enhance your security and loss prevention strategies, consider the following:
- Implement facial recognition to identify known shoplifters
- Deploy smart analytics for customer flow optimization
- Install automated POS integration for theft prevention
- Set up instant mobile alerts for security incidents
Industrial Monitoring Systems
Industrial facilities have revolutionized their monitoring capabilities through AI-powered surveillance systems that optimize production workflows while maintaining stringent safety protocols.
You can implement deep learning engines to enhance object detection and automate incident response times. These systems provide significant observations into operational efficiency, while remote control features let you manage multiple facilities from centralized locations.
ROI and Performance Metrics
Success measurement within AI-powered surveillance platforms requires careful tracking of specific performance metrics that directly impact your return on investment.
You'll need to monitor video quality, system uptime, and detection accuracy to enhance your security operations. By analyzing these metrics, you can justify investment costs and make data-driven decisions for continuous monitoring improvements.
To ensure comprehensive evaluation, consider the following key performance indicators:
- Detection accuracy rates compared to false positives/negatives
- System response time and real-time alert efficiency
- Storage optimization and bandwidth utilization
- Integration effectiveness with existing security infrastructure
Track these metrics monthly to guarantee your AI surveillance system delivers the expected ROI while maintaining peak performance levels for your security needs.
Real-world validation of these metrics comes from our V.A.L.T implementation, where success is measured through:
- Seamless scalability across 450+ organizations
- Rapid user adoption with minimal training requirements
- Efficient data management through automated scheduling and retention policies
- Enhanced operational efficiency through features like CD burning for evidence-sharing
Case Study: V.A.L.T - Advanced Video Surveillance for Specialized Industries
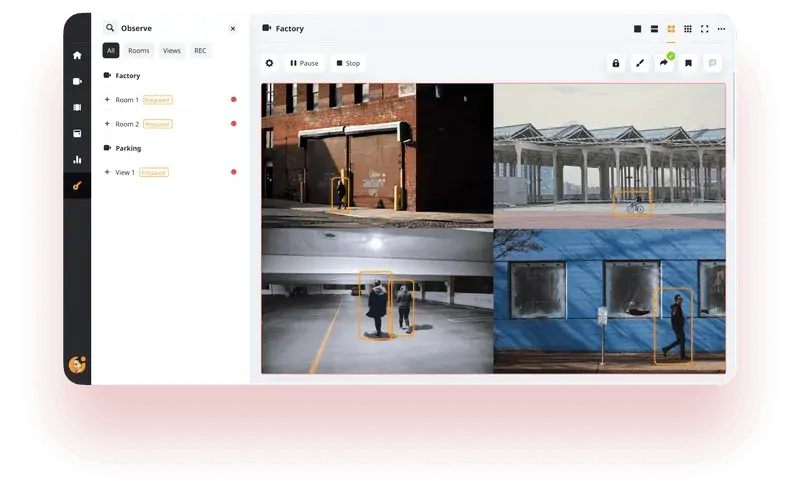
Our experience in developing V.A.L.T, a comprehensive video surveillance solution, demonstrates the practical implementation of AI-powered security systems in specialized sectors. This system serves over 450 organizations, including police departments, medical education institutions, and child advocacy centers, each with unique surveillance requirements.
When developing V.A.L.T, we focused on creating a solution that balances sophisticated functionality with user-friendly operation. The system supports HD video streaming from multiple IP cameras with advanced pan-tilt-zoom capabilities, while maintaining perfect audio-video synchronization - a crucial feature for interrogation recordings and medical training sessions.
Key implementation insights from the V.A.L.T project include:
- Security-First Design: Implementation of SSL and RTMPS encryption for data protection, coupled with LDAP integration for robust access control
- Scalable Architecture: Support for unlimited users and cameras without requiring extensive IT resources
- Intelligent Automation: Schedule-based recording and camera adjustments for efficient operation
- Enhanced Documentation: Integration of marking systems for quick reference and searchable content
This real-world application demonstrates how AI-powered surveillance can be tailored to specific industry needs while maintaining high-security standards and user accessibility.
Future Developments in AI Surveillance
To enhance your surveillance software's capabilities, you'll want to integrate emerging AI technologies like advanced facial recognition algorithms, behavioral pattern analysis, and real-time object tracking frameworks. The implementation of AI technologies has shown significant potential in enhancing operational insights and satisfaction metrics across various applications (Noranee & Othman, 2023).
You can improve system performance by implementing edge computing solutions that process data locally, reducing latency and bandwidth requirements.
Market projections indicate substantial growth opportunities in AI surveillance development, particularly in customizable solutions that prioritize privacy compliance while maintaining strong security features. This aligns with research demonstrating robust opportunities for businesses to leverage AI technologies for competitive advantage in the market (Noranee & Othman, 2023).
Emerging Technologies and Trends
Integrating AI-powered drones with your surveillance system expands monitoring capabilities while reducing the need for fixed camera installations. You'll enhance your system's effectiveness by connecting these drones to IoT sensors, creating a dynamic network that can track movement patterns and respond to environmental triggers.
The combination of aerial mobility and IoT connectivity lets you develop more flexible security solutions that adjust to changing surveillance requirements while maintaining consistent coverage.
Drone Integration
Modern AI surveillance systems have expanded beyond traditional fixed cameras through strategic drone integration, offering product owners unprecedented flexibility in their security solutions.
You can enhance your surveillance capabilities by incorporating drones that collect real-time video signals and utilize predictive analytics for proactive security measures in smart cities.
To effectively integrate these drones, consider the following steps:
- Implement automated flight paths and scheduling for continuous aerial monitoring.
- Develop real-time data synchronization between drones and central security systems.
- Create versatile AI algorithms for drone-based threat detection and tracking.
- Design user-friendly interfaces for drone fleet management and video analysis
IoT Connectivity
While surveillance technologies continue to evolve, IoT connectivity stands as a cornerstone for next-generation AI surveillance systems. You'll need to integrate your surveillance platform with cloud technology to enable seamless communication between smart devices.
Consider implementing neural networks that can process data from multiple mobile surveillance systems, allowing your platform to analyze and respond to security events in real time.
Market Growth Projections
The market contribution of AI-enhanced security systems continues to grow as organizations prioritize operational efficiency and address emerging security threats. Technological advancements and widespread adoption of cloud technology are driving this expansion, making AI surveillance more accessible and cost-effective for businesses of all sizes.
This increased accessibility is fueling a wave of development in key areas, including:
- Implement machine learning algorithms for real-time threat detection and automated alerts.
- Integrate cloud-based storage solutions with scalable architecture
- Develop APIs for seamless third-party security system integration
- Add features for automated system health monitoring and predictive maintenance
Our experience with V.A.L.T demonstrates this market evolution. What began as a straightforward video surveillance solution has grown to incorporate sophisticated features like automated scheduling, multi-camera synchronization, and intelligent access control systems, serving diverse sectors from law enforcement to healthcare education.
Practical Implementation Guide
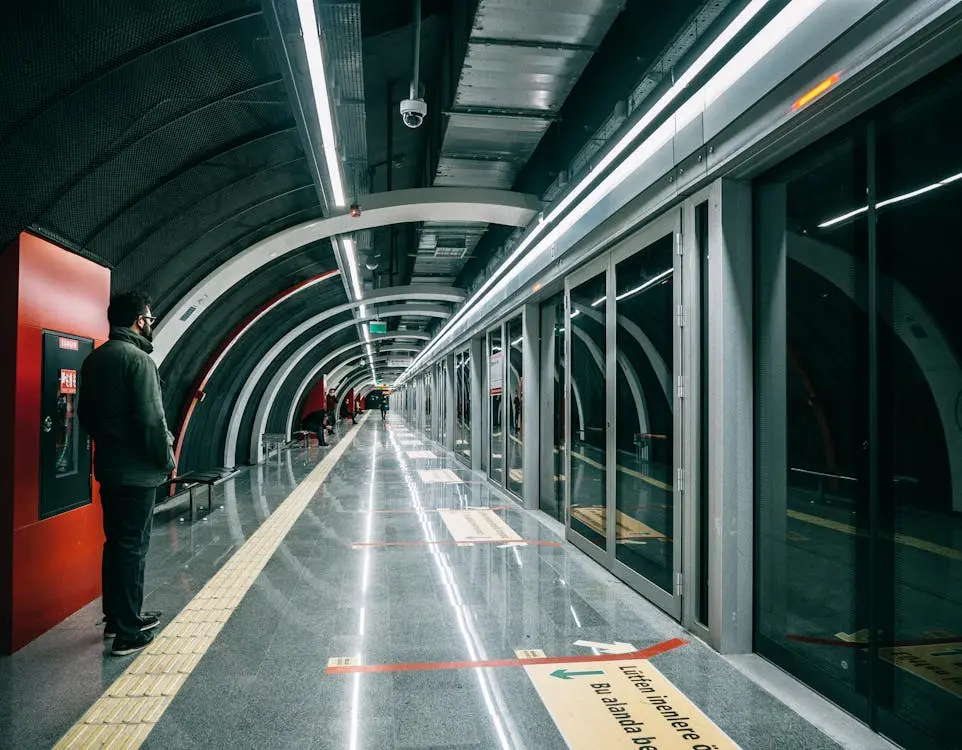
When selecting AI-powered surveillance cameras, you'll want to focus on key software capabilities like object detection accuracy, processing speed, and API compatibility with your existing systems. You can optimize your deployment by implementing a phased rollout approach, starting with high-priority areas before expanding to full coverage.
For seamless integration, make sure your development team establishes clear data-handling protocols and implements strong error-handling mechanisms in the surveillance software stack.
Selection Criteria for AI Cameras
Selecting the right AI-powered surveillance cameras requires careful consideration of both technical specifications and practical implementation factors. When evaluating options, you'll need to assess how these systems integrate with existing access control systems while minimizing security risks and human error.
Modern AI cameras have become an essential tool for enhancing security infrastructure.
- Software development capabilities, including API accessibility and SDK availability for custom integration
- Real-time processing features with support for edge computing and local analysis
- Integration flexibility with existing development frameworks and platforms
- Scalability options for future software updates and feature expansion through over-the-air updates
Based on our V.A.L.T implementation experience with industry-standard cameras like Axis, key selection factors should include:
- Integration capabilities with existing security infrastructure
- Support for high-definition streaming with minimal latency
- Pan-tilt-zoom functionality for remote operation
- Reliable audio-video synchronization capabilities
Deployment Best Practices
Regular training sessions for your development team should cover both AI model maintenance and performance monitoring to guarantee your surveillance system operates at peak efficiency.
You'll need to establish clear protocols for data validation, model retraining intervals, and system updates that align with your product's specific use cases and performance requirements.
Building a thorough maintenance schedule that includes automated testing pipelines, regular code reviews, and systematic performance benchmarking will help maintain the reliability of your AI surveillance features while minimizing technical debt.
Training Requirements
The successful deployment of AI-powered surveillance systems hinges on thorough training protocols for both the AI models and end users.
When implementing smart city development initiatives, you'll need to guarantee security professionals understand system capabilities and response efficiency requirements.
- Develop structured learning paths for AI model training
- Create user-specific training modules for different access levels
- Implement hands-on simulation exercises
- Establish continuous feedback loops for system optimization
Through our V.A.L.T deployments, we've found that intuitive interface design significantly reduces training requirements. Our modular approach allows organizations to train new users in as little as 10 minutes, focusing only on features relevant to their role.
Maintenance Protocols
Maintaining AI-powered surveillance systems requires a systematic approach to guarantee peak performance and reliability throughout their lifecycle. You'll need to regularly update edge AI cameras' software to optimize motion detection and intrusion detection capabilities. Set up automated diagnostics to monitor system health, and configure instant alerts for potential maintenance issues. Schedule periodic firmware updates to patch security vulnerabilities and enhance performance.
For instance, in V.A.L.T's implementation across medical institutions and police departments, we established:
- Automated recording schedules to reduce manual intervention
- Built-in reporting tools for system usage analytics
- Streamlined data retention management
- Regular security protocol updates
Why Trust Our AI Surveillance Expertise?
With over 19 years of specialized experience in multimedia development and AI integration, we've been at the forefront of transforming traditional surveillance systems into intelligent security solutions. Our team has successfully implemented AI-powered video surveillance platforms for over 450 organizations across law enforcement, healthcare education, and child advocacy sectors, demonstrating our deep understanding of both the technical requirements and industry-specific challenges.
Our expertise is particularly evident in our flagship V.A.L.T system, which showcases our ability to handle complex multi-camera operations while maintaining perfect AV synchronization and implementing advanced security protocols. We maintain a 100% project success rating on Upwork, reflecting our commitment to delivering reliable, scalable AI surveillance solutions that meet specific industry needs.
As specialists focused exclusively on video surveillance, e-learning, and telemedicine, we bring concentrated expertise in AI recognition, generation, and recommendations. Our rigorous team selection process (accepting only 1 in 50 candidates) ensures that every project benefits from top-tier technical knowledge in WebRTC, LiveKit, and other crucial video streaming technologies.
Frequently Asked Questions
Can Existing IP Cameras Be Upgraded With AI Capabilities Through Software Updates?
You can upgrade many IP cameras with AI through software updates, but it depends on your camera's processing capability and firmware support. Cloud-based AI solutions offer an alternative to older hardware.
What Programming Languages Are Recommended for Developing AI Camera Applications?
You'll want to use Python for AI development, with frameworks like TensorFlow and OpenCV. JavaScript and Node.js work well for web interfaces and cloud connectivity.
How Can Developers Optimize AI Models for Edge Computing in Cameras?
You'll need to quantize your models, prune unnecessary layers, and use TensorFlow Lite or ONNX for compression. Consider model distillation and hardware-specific optimizations to maximize edge device performance.
What APIS Are Available for Integrating Third-Party AI Solutions?
You'll find strong APIs like Google Cloud Vision, Amazon Rekognition, and Azure Computer Vision for AI integration. OpenCV and TensorFlow also offer flexible APIs for custom model deployment and inference.
How Do You Handle Version Control for AI Model Updates Across Camera Networks?
You'll need to implement a central model registry to track versioning, use container orchestration for deployments, and automate rollbacks. Consider using tools like MLflow or DVC for AI model version control.
How Can Organizations Ensure Smooth Integration of AI Surveillance Across Multiple Facilities?
Based on our experience with V.A.L.T's deployment across 450+ organizations, successful multi-facility integration requires:
- Browser-based accessibility for easy deployment
- Scalable architecture supporting unlimited users and cameras
- Centralized management of user permissions and access controls
- Standardized protocols for data handling and security
To Sum Up
You'll need to carefully plan your AI implementation strategy to successfully transform your IP cameras into intelligent surveillance systems. As demonstrated through our V.A.L.T implementation across diverse sectors, the key to success lies in balancing advanced functionality with user accessibility. Whether you're serving law enforcement, healthcare education, or child advocacy organizations, focus on creating scalable infrastructure, implementing strong security measures, and maintaining intuitive interfaces. Start with core capabilities and gradually expand based on specific industry needs while ensuring data privacy and compliance at every step.
Final Thoughts: Don't let your surveillance system fall behind in the AI revolution.
At Fora Soft, our expertise in video surveillance and AI integration is demonstrated through successful projects like V.A.L.T, which serves over 450 client organizations with state-of-the-art surveillance solutions.
Schedule a consultation today to discuss your project, or explore our portfolio to see how we've helped others transform their security systems.
References:
Gadal, S., Mokhtar, R., & Abdelhaq, M., et al. (2022). Machine learning-based anomaly detection using k-mean array and sequential minimal optimization. Electronics., 11(14), 2158. https://doi.org/10.3390/electronics11142158
Ke, R., Zhuang, Y., Pu, Z., & Wang, Y. (2021). A smart, efficient, and reliable parking surveillance system with edge artificial intelligence on IoT devices. Transactions on Intelligent Transportation Systems, 22(8), pp. 4962-4974. https://doi.org/10.1109/tits.2020.2984197
Kumar, S. (2023). Artificial intelligence. Journal of Computer Mechanics and Management, 2(3), pp. 31-42. https://doi.org/10.57159/gadl.jcmm.2.3.23064
Noranee, S., & Othman, A. (2023). Understanding consumer sentiments: Exploring the role of artificial intelligence in marketing. Journal of Economics and Management, 10(1), pp. 15-23. https://doi.org/10.30996/jmm17.v10i1.8690
Ong, A. (2023). IP cameras beyond security: A systematic literature review examining their adoption and potential in the healthcare industry. International Journal of Membrane Science and Technology, 10(1), pp. 936-944. https://doi.org/10.15379/ijmst.v10i1.2687
Satyasree, E. (2023). Edge AI for real-time video analytics in surveillance systems. International Journal on Recent and Innovation Trends in Computing and Communication, 11(10), pp. 2269-2275. https://doi.org/10.17762/ijritcc.v11i10.8947
Comments