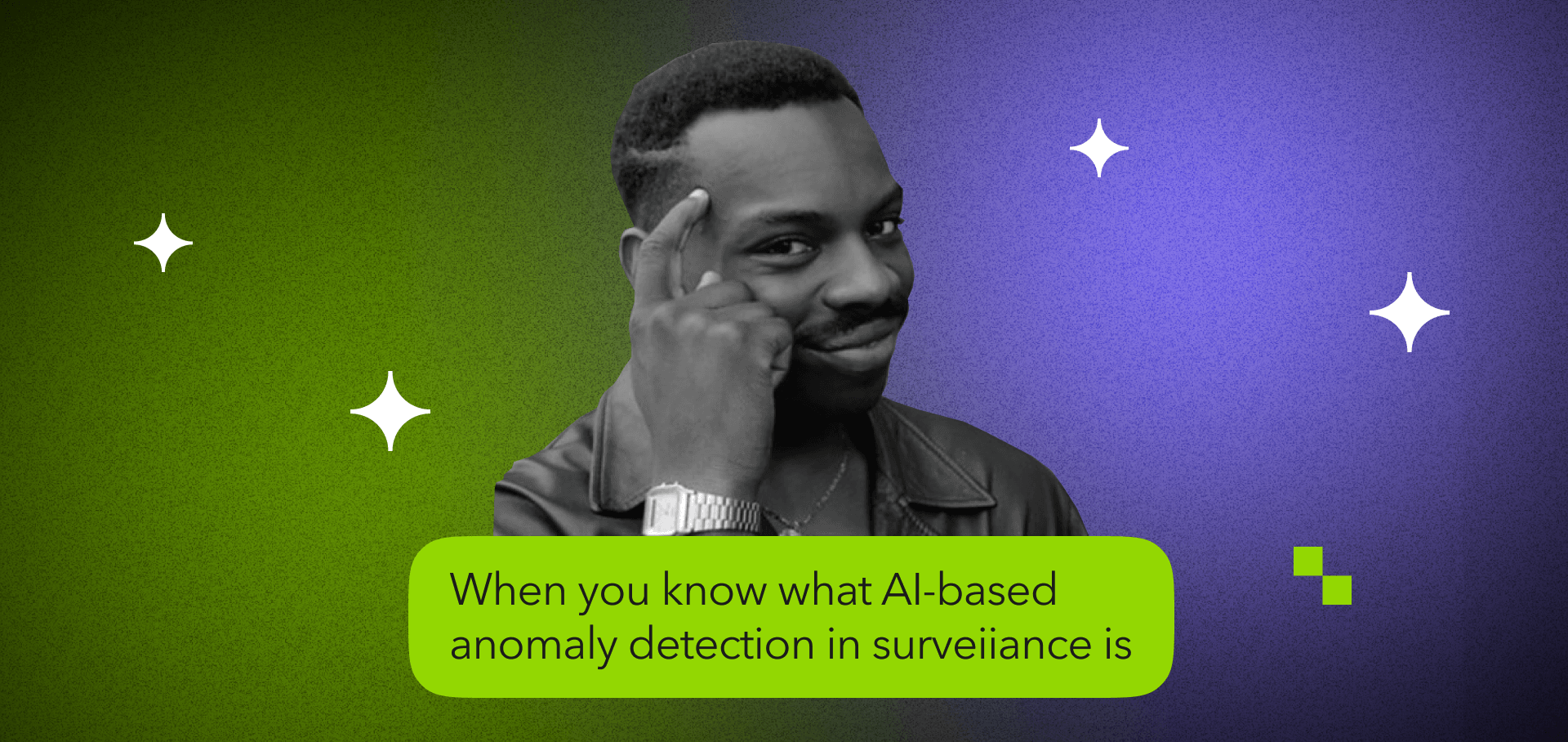
As you explore the field of AI-based anomaly detection in surveillance, you'll discover how this cutting-edge technology is revolutionizing public safety and security. By utilizing advanced algorithms and real-time data analysis, these systems can identify unusual patterns and behaviors that might otherwise go unnoticed. From detecting unauthorized access to pinpointing abnormal activities, AI-based anomaly detection is becoming an essential tool in the fight against crime and potential threats.
But how exactly does it work, and what are the key components that make this technology so powerful? Let's take a closer look at the fascinating field of AI-based anomaly detection.
Key Takeaways
- AI-based anomaly detection in surveillance identifies suspicious activities, abnormal behavior, and security risks in video feeds using machine learning algorithms
- It involves data collection, preprocessing, feature selection, model training, real-time monitoring, and establishing baselines of normal behavior patterns
- Continuous analysis and feedback loops improve understanding of normal behavior, track activities, identify deviations, and anticipate future incidents
- Integration with existing cloud video security systems, edge computing for fast processing, and ensuring security and privacy compliance are crucial
- Challenges include limited labeled data, reducing false positives, and adapting to changing patterns in behaviors and environments
Importance of Anomaly Detection
Spotting unusual patterns in surveillance footage is essential for maintaining public safety and preventing potential threats. You can identify suspicious activities, abnormal behavior, and potential security risks by detecting anomalies in video feeds. This allows you to respond quickly to incidents, mitigate dangers, and guarantee the well-being of monitored individuals and assets.
Anomaly detection forms a critical component of modern security infrastructure, enabling you to sift through vast amounts of surveillance data to pinpoint concerning events that require further investigation or immediate action. Integrating anomaly detection capabilities into surveillance systems equips you to stay one step ahead of potential threats and maintain a safer environment.
Core Components
When it comes to the core components of AI-based anomaly detection in surveillance, you'll need to focus on two key aspects. First, there's the essential step of data collection and preprocessing, which involves gathering relevant surveillance data and preparing it for analysis.
Additionally, feature selection plays a significant role in training the AI system to accurately identify anomalies, as you must choose the most informative and discriminative features to optimize performance.
Data Collection and Preprocessing
You'll need an extensive, diverse dataset to train your AI model for accurate anomaly detection in surveillance footage. Collecting vast amounts of video data from various sources is essential. This includes normal footage as well as examples of anomalies you want to detect. Once you've gathered the data, preprocessing involves extracting key features from the raw video frames. These spatiotemporal features capture vital information about objects, movements, and interactions over time.
Through feature engineering, you'll transform the extracted features into a more representative format that the AI model can effectively learn from. Preprocessing may also include data cleaning, normalization, and augmentation techniques to enhance the quality and diversity of your training dataset.
Feature Selection for Training
Through careful feature selection, you can identify the most discriminative and informative attributes that enable your AI model to effectively distinguish between normal and anomalous behavior in surveillance footage.
To create a robust anomaly detection system, you need to select the most relevant features from your training videos. These features may include appearance features, such as object size, shape, and color, as well as motion features, like speed, direction, and trajectory. By focusing on the most discriminative features, you can train models that accurately identify examples of anomalies, such as loitering, vandalism, or theft. Feature selection is a vital step in building an effective AI-based anomaly detection system, as it directly impacts the model's ability to learn and generalize from the training data.
Model Training and Monitoring
To effectively train and monitor AI models for anomaly detection in surveillance, you'll need to take into account both supervised and unsupervised learning approaches. You should also implement real-time monitoring to guarantee the models continue to perform well on live data streams. It's vital to regularly evaluate and update the models as new data becomes available to maintain their accuracy and reliability over time.
Supervised and Unsupervised Learning
When training AI models for anomaly detection in surveillance, you can utilize either supervised or unsupervised learning techniques, each with its own advantages and considerations for model monitoring. Supervised learning involves training the model on labeled data, where normal and abnormal patterns are pre-defined. This approach allows for more targeted anomaly detection but requires extensive data labeling.
On the other hand, unsupervised learning enables the model to identify anomalies without prior labeling by learning the inherent patterns in the data. Deep learning techniques, such as autoencoders or generative adversarial networks, are commonly used in unsupervised anomaly detection algorithms.
When monitoring the performance of these models, consider the following:
- Regularly assess the model's accuracy and false positive/negative rates
- Adjust to evolving normal patterns and emerging anomalies
- Continuously update and retrain the model with new data
Real-Time Monitoring
For effective real-time monitoring in AI-based anomaly detection for surveillance, you'll need to implement continuous model training and performance tracking. Video anomaly detection software should be designed to provide real-time alerts when abnormal events or unusual activities are detected. Continuous monitoring is essential to guarantee that the system remains up-to-date and accurately identifies anomalies as they occur.
By constantly feeding new data into the model, you can improve its ability to identify patterns and detect deviations from the norm. This ongoing training process helps the system adjust to changing environments and maintain ideal performance over time. Real-time monitoring also enables swift responses to potential threats, allowing security personnel to take immediate action when necessary to prevent or mitigate incidents.
Establishing Baselines
To establish meaningful baselines for anomaly detection, you need to understand normal behavior patterns in your surveillance environment. You should continuously analyze surveillance data, looking for consistent trends and common activities.
By incorporating feedback loops, you can refine your baselines over time as you gain more data and understanding into typical behaviors.
Normal Behavior Patterns
Establishing normal behavior patterns is the foundation for detecting anomalies in AI-based surveillance systems. By analyzing vast amounts of data from real-time monitoring, these systems can identify complex patterns that represent typical activities in a given environment. This allows the AI to create a baseline of expected behavior, which serves as a reference point for detecting unusual events.
Some common examples of normal behavior patterns include:
- Regular foot traffic in public spaces during peak hours
- Vehicles following established traffic patterns and parking norms
- Employees accessing secure areas with proper credentials
When the AI detects deviations from these established norms, it can flag them as potentially anomalous events for further investigation, helping to enhance security and efficiency in surveillance operations.
Continuous Analysis and Feedback Loops
AI-based surveillance systems continuously analyze data and employ feedback loops to refine their understanding of normal behavior patterns over time. They use real-time monitoring and advanced analytics to track activities, identifying deviations that could indicate potential threats or issues. As anomaly detection models process more data, they become better at spotting unusual events. Predictive analytics helps anticipate future incidents based on historical patterns.
When anomalies are detected, the system can trigger alerts for human operators to review and take corrective actions if needed. This feedback is then incorporated back into the models, fine-tuning their accuracy. Through ongoing learning and adjustment, AI-based anomaly detection grows increasingly effective at keeping people and assets safe. The cycle of analysis, detection, response, and refinement never stops.
Integration with Technology
When integrating AI-based anomaly detection into your surveillance system, you'll need to verify compatibility with existing hardware and software components. To enable real-time processing of video feeds, consider deploying edge computing devices that can analyze data locally, reducing latency and bandwidth requirements. Additionally, it's vital to comply with relevant security and privacy regulations, such as GDPR or HIPAA, depending on your industry and jurisdiction.
System Compatibility
To guarantee seamless integration with existing surveillance infrastructure, you'll need to contemplate the compatibility of AI-based anomaly detection systems with current hardware and software components.
Consider these key aspects:
- Make sure your AI-driven anomaly detection system is compatible with existing cloud video security systems and surveillance monitoring setups
- Verify that the system can handle real-time anomaly detection and integrate with various vision applications
- Check that your hardware meets the processing power and storage requirements for smooth operation
Edge Computing for Fast Processing
Utilize edge computing to enable rapid, on-site processing of video data for real-time anomaly detection. By utilizing deep learning-based techniques directly on surveillance devices, you can avoid the latency and bandwidth issues associated with cloud processing. This allows AI-driven anomaly detection technology to analyze video feeds as they're captured, identifying potential threats or unusual behavior patterns almost instantly.
However, it's important to guarantee that the edge devices you deploy have sufficient computational power to handle these complex tasks without introducing performance issues. When implemented correctly, edge computing can greatly enhance the effectiveness of your surveillance system, enabling security personnel to respond quickly and initiate timely intervention when necessary.
Security and Privacy Compliance
Integrating AI-based anomaly detection into your surveillance system requires careful consideration of security and privacy compliance. You must guarantee that your system is protected against cyber threats and potential security breaches.
Here are some key points to keep in mind:
- Implement robust encryption and access controls to safeguard sensitive data
- Regularly update software and firmware to address vulnerabilities
- Establish clear policies and procedures for handling and sharing data with law enforcement
Applications
There are several key applications for AI-based anomaly detection in surveillance systems. You can use it to detect intrusions, such as unauthorized people entering restricted areas. It's also significant for monitoring behavior and spotting unusual activities that may indicate a security threat, as well as identifying equipment failures before they lead to bigger problems.
Intrusion Detection
AI-powered anomaly detection systems frequently play an essential role in intrusion detection applications, alerting security personnel to potential threats in real-time. These unified AI video security solutions excel at human detection, identifying unauthorized individuals who enter restricted areas. Our V.A.L.T. project exemplifies this technology, offering a scalable and sophisticated approach to video surveillance.
AI video security solutions can also spot strange actions that deviate from normal behavior patterns, such as:
- Loitering in sensitive zones
- Attempting to access locked doors or windows
- Moving erratically or suspiciously
Behavioral Anomaly Monitoring
Beyond intrusion detection, AI-driven behavioral anomaly monitoring finds important applications across various fields, enhancing safety, efficiency, and user experiences. AI-powered anomaly detection systems can analyze human behavior patterns in real-time, identifying deviations from the norm.
In retail settings, these systems can detect shoplifting attempts or suspicious activities, alerting security personnel promptly. Anomaly detection also plays an essential role in fraud prevention, especially in financial institutions, by flagging unusual transactions or account activities.
In healthcare, AI can monitor patient behavior and critical signs, detecting anomalies that may indicate potential health issues. Real-world applications of behavioral anomaly monitoring extend to manufacturing, where AI can identify equipment malfunctions or process deviations, enabling proactive maintenance and minimizing downtime.
Equipment Failure Detection
Machine learning models, such as neural networks, are trained on historical data to identify normal equipment behavior patterns. When deployed, these models continuously monitor real-time data from sensors and cameras, comparing it against expected norms. If deviations exceed predefined thresholds, the anomaly detection software triggers alerts for investigation.
Benefits of AI-based equipment failure detection include:
- Reduced unplanned downtime and maintenance costs
- Increased equipment reliability and lifespan
- Improved safety and compliance
Challenges
When implementing AI-based anomaly detection in surveillance, you'll face several challenges. One major hurdle is the limited availability of labeled data, which can hinder the training and performance of AI models. Additionally, reducing false positives while maintaining high detection accuracy is a constant balancing act that requires fine-tuning and optimization.
Limited Labeled Data
One of the key challenges in developing AI-based anomaly detection systems for surveillance is the scarcity of labeled data. Since anomalies are rare by definition, it's difficult to collect enough examples to train a deep learning model effectively.
This limited labeled data makes it harder to:
- Capture the full range of potential anomalies
- Train models to detect subtle anomalies that may be easy to miss
- Avoid false positives from normal behaviors that resemble outliers
To address this, researchers often turn to unsupervised learning techniques for outlier detection. These methods can learn patterns from unlabeled data to flag unusual events. However, the lack of labels still constraints performance, so expanding datasets with simulations and annotations is an active area of research.
Reducing False Positives
Alongside the challenge of limited labeled data, false positives pose another considerable hurdle in AI-based anomaly detection for surveillance. False positives occur when the system incorrectly identifies patterns indicative of potential anomalies, leading to unnecessary alerts and reduced accuracy of anomaly detection. This can result in 'alert storms,' overwhelming security personnel with a flood of false alarms and making it difficult to identify genuine threats.
To minimize false positives, AI models must be trained on diverse and representative data, allowing them to better distinguish between normal and abnormal behavior. Additionally, implementing advanced techniques such as flexible thresholding and context-aware analysis can help refine the system's decision-making process. By reducing false positives, AI-based anomaly detection can provide more reliable and actionable observations for effective surveillance.
Adapting to Changing Patterns
In AI-based anomaly detection for surveillance, adjusting to evolving patterns presents a considerable challenge. As behaviors and environments change over time, the model for anomaly detection must be modified to maintain accuracy in identifying abnormal events. Without this capability, the system's effectiveness in real-world anomaly detection diminishes.
To address this issue, researchers are developing advanced techniques for domain video anomaly detection that can:
- Continuously learn and update the definition of normal behavior
- Quickly detect and modify to new patterns of anomalies
- Maintain a high level of performance despite shifts in the monitored environment
Frequently Asked Questions
How Does AI-Based Anomaly Detection Differ From Traditional Methods?
AI-based anomaly detection is more advanced than traditional methods. It uses machine learning algorithms to identify patterns and detect unusual behavior in real-time, while traditional methods rely on predefined rules and thresholds.
What Types of Anomalies Can AI-Based Systems Detect in Surveillance Footage?
AI-based systems detect unusual behaviors, suspicious objects, crowd anomalies, and safety violations in surveillance footage. They're trained to spot patterns that deviate from the norm, alerting security personnel to potential threats.
How Accurate Are AI-Based Anomaly Detection Systems in Real-World Scenarios?
AI-based anomaly detection systems can be quite accurate in real-world scenarios, but they're not perfect. Factors like camera placement, lighting conditions, and the intricacy of the scene can impact their effectiveness.
What Is the Role of Human Operators in AI-Based Anomaly Detection?
Human operators play an essential role in AI-based anomaly detection. They're responsible for configuring the system, monitoring alerts, and verifying detected anomalies. Their judgment is vital for determining which events require further investigation or response.
How Can Organizations Ensure the Privacy and Security of Surveillance Data?
To protect privacy and security, organizations should encrypt surveillance data, limit access to authorized personnel, and regularly audit your systems. Implement clear data retention policies and be transparent about your practices with employees and customers.
To sum up
You now have a solid understanding of AI-based anomaly detection in surveillance. It's a powerful tool for enhancing security, utilizing advanced algorithms to identify unusual patterns and potential threats in real-time. While challenges exist, such as data quality and privacy concerns, the applications are vast, ranging from public safety to infrastructure monitoring. As technology continues to evolve, AI-driven anomaly detection will play an increasingly vital role in strengthening surveillance systems and keeping communities safe.
You can find more about our experience in AI development and integration here
Interested in developing your own AI-powered project? Contact us or book a quick call
We offer a free personal consultation to discuss your project goals and vision, recommend the best technology, and prepare a custom architecture plan.
Comments